Employee Training Management AI - Boost Skills Effortlessly
50% of organizations are now using AI for training and development, reflecting rapid growth in adoption since 2023, according to HireBee. This skills revolution demands a fundamental shift in how companies approach employee training. Enter Employee Training Management AI – a revolutionary approach that transforms corporate learning from a standardized, one-size-fits-all experience into a dynamic, personalized journey tailored to each employee's unique needs and learning style.
Unlocking the Potential of AI in Employee Training Management
The traditional approach to employee training—characterized by generic content delivery and scheduled classroom sessions—is rapidly becoming obsolete in today's fast-paced business environment. AI technology offers a compelling alternative, creating learning experiences that adapt to individual needs while reducing administrative burdens for training managers.
Understanding AI's Role in Modern Employee Training
The integration of artificial intelligence in training and development represents a paradigm shift in corporate learning strategies. As organizations face increasing pressure to upskill their workforce efficiently, AI emerges as a critical enabler of more effective, engaging, and personalized training experiences.
What is Employee Training Management AI?
Employee Training Management AI refers to the application of artificial intelligence technologies to enhance, automate, and optimize corporate learning initiatives. This technology leverages advanced algorithms and machine learning capabilities to create more effective training experiences by analyzing employee performance data, identifying skill gaps, and delivering tailored content.
AI in training and development encompasses various technologies including natural language processing for content analysis, machine learning algorithms for personalization, and predictive analytics for identifying future skill requirements. These systems continuously learn from interactions, improving their recommendations and interventions based on empirical data rather than assumptions.
As Clara Shih, CEO of Salesforce AI, notes: "Right now, people talk about being an AI company… but it'll be unthinkable not to have intelligence integrated into every product and service. It'll just be an expected, obvious thing."
How AI is Transforming Training Programs
AI is fundamentally reshaping how organizations develop and deliver training content. By analyzing vast amounts of data on employee performance, learning patterns, and content engagement, AI systems can identify which training methodologies are most effective for specific roles, departments, or individual learning styles.
Adaptive learning platforms powered by AI dynamically adjust content difficulty based on learner progress, ensuring employees are consistently challenged without becoming overwhelmed. This stands in stark contrast to traditional approaches where all participants progress through identical material at the same pace regardless of their existing knowledge or learning capacity.
Furthermore, AI enables real-time feedback mechanisms that provide immediate guidance rather than delayed assessment. For example, virtual role-play simulations utilizing AI can evaluate communication techniques, offering instantaneous coaching on areas for improvement without the need for human facilitators to be present.
Why Integrate AI into Employee Training?
Organizations increasingly recognize that competitive advantage in the digital economy hinges on workforce capabilities and agility. AI-powered training solutions address this imperative by accelerating skill development while optimizing resource allocation.
Benefits of AI-driven Training Solutions
The implementation of artificial intelligence in training and development delivers multiple tangible advantages. Training efficiency improves significantly through automated content curation, which matches learning materials to specific skill gaps without requiring manual intervention from learning and development teams.
Knowledge retention rates typically increase when training is personalized, as employees engage more deeply with content relevant to their specific roles and learning preferences. AI-driven training increases employee engagement by 72%, while teams using AI tools complete projects 33% faster and with 26% fewer resources, demonstrating significant improvements in productivity and efficiency.
From a financial perspective, AI-driven training often delivers superior return on investment. Marketing teams leveraging AI tools generate 38% more qualified leads, while financial analysis using AI techniques leads to 29% more accurate forecasting—directly contributing to improved business outcomes.
Challenges and Considerations
Despite its transformative potential, implementing AI in training management presents several challenges. Data quality remains paramount—AI systems require substantial, accurate data to generate meaningful insights and recommendations. Organizations with limited learning data may need to build their datasets before realizing the full benefits of AI-powered training.
Ethical considerations also demand attention, particularly regarding algorithmic bias. As Rumman Chowdhury, CEO of Parity AI, emphasizes: "Responsible AI is not just about liability — it's about ensuring what you are building is enabling human flourishing." Training algorithms must be carefully designed and monitored to ensure they don't perpetuate existing biases in career development or learning opportunities.
Finally, successful AI implementation requires change management strategies that prepare both training professionals and employees for new learning methodologies. Resistance often stems from concerns about technology replacing human interaction in the learning process—a legitimate consideration that requires balancing automated elements with opportunities for social learning and human coaching.
{{cta}}
Designing Effective AI-Enhanced Training Programs
Creating impactful AI-powered training programs requires a strategic approach that balances technological innovation with fundamental learning principles. The most successful implementations don't simply deploy AI training software as a technological overlay to existing methods, but rather reimagine the learning experience from the ground up with AI capabilities as a foundational element.
Personalization: Tailoring Training to Individual Needs
Personalization represents one of the most powerful applications of AI tools for training and development. Unlike traditional one-size-fits-all approaches, AI-driven personalization analyzes individual employee data—including performance metrics, learning history, career trajectory, and even behavioral patterns—to create uniquely tailored learning pathways.
Advanced AI training tools can identify specific knowledge gaps by comparing an employee's demonstrated skills against role requirements or organizational competency frameworks. For instance, an AI system might recognize that a customer service representative excels at product knowledge but requires development in conflict resolution techniques. The system then prioritizes relevant conflict management content while reducing time spent on already-mastered product information.
This efficiency gain stems from eliminating redundant content while focusing exclusively on relevant skill development areas, creating a more engaging and meaningful learning experience for each employee.
Adaptive Learning: Adjusting to Progress in Real-Time
Adaptive learning represents the dynamic counterpart to personalization, focusing on real-time adjustments rather than pre-planned pathways. AI training software continuously monitors learner progress, analyzing performance patterns to make instantaneous adjustments to content difficulty, learning pace, and instructional approaches.
This capability proves particularly valuable for complex skill development where employees may progress unevenly across different competency areas. For example, an adaptive learning system teaching data analysis might identify that a learner quickly masters visualization concepts but struggles with statistical interpretation. The system automatically allocates additional practice opportunities and explanatory content for statistical concepts while accelerating through visualization modules.
The technical foundation for effective adaptive learning relies on sophisticated algorithmic decision trees combined with machine learning capabilities that improve recommendations over time. Leading AI training tools incorporate reinforcement learning techniques that optimize instructional pathways based on observed outcomes across thousands of learners, continuously refining the adaptive response mechanisms.
Content Variety: Mixing AI Tools with Traditional Methods
Despite AI's powerful capabilities, research consistently demonstrates that blended learning approaches—combining technological and traditional methodologies—yield superior outcomes compared to either approach in isolation. Effective AI-enhanced training programs strategically integrate various learning modalities based on content requirements and learning objectives.
AI training software excels at delivering certain types of training content—particularly standardized knowledge transfer, procedural learning, and skills practice through simulation. Conversely, traditional instructor-led sessions often prove more effective for complex conceptual discussions, cultural transformation initiatives, and scenarios requiring nuanced interpersonal dynamics.
Organizations implementing successful blended programs leverage AI for pre-training assessments, knowledge foundations, and post-training reinforcement while utilizing human-led sessions for collaborative problem-solving, contextual application, and values-based discussions. This combination maximizes the strengths of both approaches while mitigating their respective limitations.
Automation: Streamlining Administrative Tasks
Beyond direct learning applications, AI tools for training and development deliver substantial organizational benefits by automating administrative functions that traditionally consume significant resources. Training administration typically requires 30-40% of training department capacity, representing a substantial opportunity for efficiency gains through automation.
AI training software can streamline numerous administrative workflows including session scheduling (with intelligent conflict resolution), prerequisite tracking, compliance monitoring, certification management, and comprehensive reporting. Advanced systems even automate content maintenance by identifying outdated materials based on usage patterns and external information sources.
Particularly valuable is AI's ability to generate actionable insights from training data that would otherwise remain hidden in conventional reporting. For example, predictive analytics can identify correlations between specific training interventions and subsequent performance improvements, enabling data-driven decisions about program investments. Similarly, AI can detect early warning signals of employee disengagement or turnover risk based on training participation patterns, providing opportunities for proactive intervention.
By transferring these administrative burdens to AI systems, learning professionals can reallocate their expertise toward higher-value activities such as strategic program design, facilitation of complex learning experiences, and personalized coaching—functions where human judgment and expertise remain irreplaceable despite technological advances.
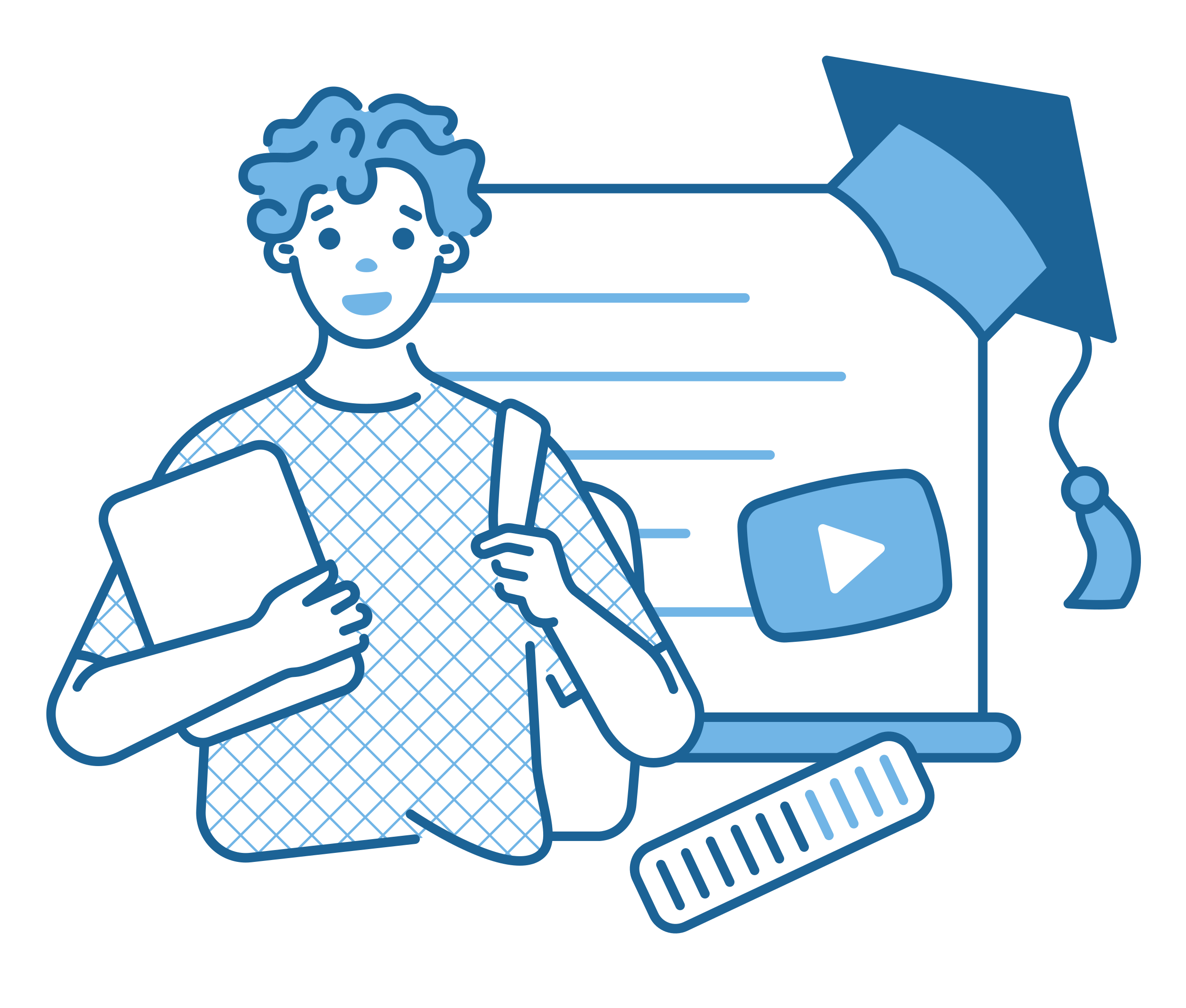
Generative AI: Transforming Content Creation and Delivery
The emergence of generative AI and large language models (LLMs) represents a significant evolution in employee training capabilities. These technologies are revolutionizing how training content is created, personalized, and delivered at scale.
How Generative AI is Reshaping Training Content
Generative AI models can rapidly produce high-quality training materials across various formats, dramatically reducing content development time while ensuring consistency. These systems analyze existing company documentation, industry best practices, and subject matter expert input to generate comprehensive training modules tailored to specific organizational contexts.
As Sam Altman, CEO of OpenAI, emphasizes: "We see the wave coming. Now this time next year, every company has to implement it — not even have a strategy. Implement it." This urgency applies particularly to training departments seeking to remain competitive in rapidly evolving skill landscapes.
Beyond simple content creation, generative AI enables dynamic scenario generation for realistic simulations. For example, customer service training can incorporate AI-generated scenarios reflecting actual customer interactions and evolving product features, ensuring relevance and applicability without requiring constant manual updates by instructional designers.
Real-World Applications of Generative AI in Training
Organizations across industries are implementing generative AI to transform their training approaches:
Personalized Learning Paths and Content
A large financial institution partnered with Correlation One to implement a customized generative AI training platform. The program began with AI readiness assessments to identify specific skill levels and use cases relevant to different business units. Through immersive learning sessions and internal competitions, the organization achieved a 25% average increase in process automation tasks completed by teams post-training.
Multilingual Training Support
Lark deployed an AI-powered suite featuring automatic transcription and instant translation capabilities to support global training initiatives. This implementation addressed language barriers and enabled consistent training experiences across diverse geographic locations. The results were significant: 50% faster onboarding and 35% improvement in material retention compared to previous non-AI methods.
AI-Powered Coaching and Feedback
A technology enterprise implemented a generative AI pilot program that utilized ChatGPT and custom LLMs integrated into internal knowledge bases and scenario simulators. The program began with a select group in product support and technical training, gradually expanding based on success metrics. The organization achieved a 20% reduction in support ticket resolution time and 80% employee satisfaction with AI-generated training materials.
Future Applications and Ethical Considerations
The potential of generative AI in training continues to expand, with emerging applications including:
- Real-time translation of training materials across languages and cultural contexts
- Dynamic content generation that adapts to emerging market trends and organizational changes
- AI-facilitated peer learning networks that connect employees based on complementary skills
- Personalized coaching simulations that provide safe spaces to practice difficult conversations
However, these advancements come with ethical responsibilities. As Rumman Chowdhury notes, responsible AI development must focus on human flourishing. Organizations must maintain transparency about AI-generated content, monitor for potential biases, and ensure that AI enhances rather than replaces meaningful human connections in the learning process.
AI Tools and Technologies for Employee Training
The landscape of employee training has undergone a dramatic transformation with the integration of artificial intelligence. Modern AI for learning and development transcends simple automation, offering unprecedented capabilities for personalization, skill assessment, and adaptive content delivery. Organizations that strategically implement these technologies gain significant competitive advantages through accelerated skill development and more engaged workforces.
Top AI Tools Revolutionizing Employee Training
The market for AI training software has expanded rapidly, with solutions addressing various aspects of the learning journey. These tools range from comprehensive platforms that manage entire training ecosystems to specialized applications focusing on specific learning challenges.
Overview of Leading Platforms
WorkRamp stands as a prominent AI-driven Learning Management System that has gained traction for its ability to create personalized learning paths based on individual skill gaps and career trajectories. The platform's adaptive algorithms continuously refine content recommendations as employees progress, ensuring material remains appropriately challenging without becoming overwhelming.
Conversational AI tools like Articulate Rise and EdCast incorporate natural language processing to provide interactive learning experiences. These platforms function as virtual teaching assistants, answering employee questions at any hour and providing just-in-time performance support that reinforces formal training. Their ability to simulate real-world conversations makes them particularly effective for soft skills development and customer service training.
Content creation has been revolutionized by AI tools for training and development such as Synthesia and Vyond, which enable training departments to generate professional-quality video content without extensive production resources. These platforms can transform text-based training materials into engaging visual presentations, complete with AI-generated narration in multiple languages—dramatically reducing the cost and time required for multilingual training development.
Learning analytics represents another crucial category where platforms like LearnUpon and Docebo excel. Their AI capabilities extend beyond basic completion tracking to provide predictive insights about skill development trajectories and identify correlations between specific learning interventions and performance improvements. This data-driven approach enables continuous optimization of training investments based on demonstrable outcomes rather than assumptions.
Case Studies Highlighting Success Stories
IBM's implementation of AI training tools demonstrates the transformative potential of these technologies at scale. The company deployed an AI-powered learning platform that analyzes employee skills, identifies gaps relative to emerging market demands, and proactively recommends personalized development paths. This approach reduced time-to-proficiency for technical roles by 37% while simultaneously increasing employee satisfaction with learning resources by 42%.
Telecommunications leader Vodafone implemented a virtual AI coaching program that provides managers with daily micro-learning experiences and personalized feedback on communication patterns. Post-implementation analysis revealed a 23% improvement in employee engagement scores under managers participating in the AI coaching program, compared to just 7% improvement in control groups using traditional training approaches.
Retail giant Walmart successfully deployed AI-powered simulation training to prepare associates for high-stress situations like Black Friday sales events. The immersive training system adapts scenarios based on individual performance, providing additional practice in areas where employees demonstrate weakness. This targeted approach reduced customer complaints during peak shopping periods by 31% while cutting training time by nearly half.

Implementing AI Solutions: A Practical Framework
Successful AI implementation requires thoughtful planning and strategic execution. Organizations achieving the greatest returns approach AI training tools as transformational initiatives rather than simply purchasing new software.
1. Assessment and Preparation
- Audit Current Training Infrastructure: Identify specific pain points and inefficiencies that AI might address
- Evaluate Data Readiness: Assess quality and availability of learning data to power AI algorithms
- Stakeholder Analysis: Identify key stakeholders and potential resistance points
- Define Clear Objectives: Establish specific, measurable goals for AI implementation
2. Selection Criteria for AI Tools
- Integration Capabilities: Ensure compatibility with existing systems
- Data Security and Privacy Features: Verify compliance with relevant regulations
- Scalability Parameters: Assess performance at projected organizational scale
- Implementation Support: Evaluate vendor change management methodologies
- User Experience Quality: Prioritize intuitive interfaces for both administrators and learners
3. Pilot Implementation Strategy
- Select Representative User Group: Choose a diverse sample of end-users
- Define Success Metrics: Establish clear KPIs for the pilot phase
- Communication Plan: Develop transparent messaging about AI capabilities and limitations
- Training for Administrators: Ensure proper configuration and management
- Feedback Mechanisms: Create channels for user input and issue reporting
4. Full Deployment Approach
- Phased Rollout Plan: Schedule gradual implementation across departments
- Technical Support Structure: Establish dedicated assistance resources
- Change Management Activities: Address resistance through education and demonstration
- Data Governance Framework: Define protocols for data collection and usage
- Integration with Existing Programs: Determine which traditional elements to maintain
5. Continuous Optimization
- Regular Performance Reviews: Schedule periodic assessment of AI effectiveness
- Update and Refinement Process: Establish protocols for system improvements
- Emerging Technology Evaluation: Monitor developments in AI training capabilities
- Expanded Use Cases: Identify additional applications as organizational comfort increases
- ROI Measurement: Continuously assess return on AI training investments
This framework provides a structured approach to implementing AI in training environments, addressing the full lifecycle from initial assessment through ongoing optimization.
{{cta}}
Evaluating and Measuring the Impact of AI in Training
Implementing AI-enhanced training solutions represents a significant investment for organizations—both financially and operationally. Without robust evaluation frameworks, however, these investments risk becoming technology experiments rather than strategic assets. Effective measurement approaches not only justify AI implementation costs but also provide the data necessary to continuously optimize training effectiveness and address the growing AI skills gap within organizations.
Setting KPIs and Metrics for Success
Establishing meaningful key performance indicators requires aligning measurement approaches with both learning objectives and broader business goals. Traditional training metrics like completion rates and satisfaction scores remain relevant but insufficient when evaluating AI-powered learning systems.
Leading organizations implement multi-dimensional measurement frameworks that capture both learning process metrics and business outcome indicators. Process metrics might include engagement levels (time spent in learning activities), completion rates, assessment performance, and learning pathway progression. These metrics help evaluate the effectiveness of the learning experience itself and identify potential friction points in the AI delivery model.
Business outcome metrics connect learning activities to tangible organizational results. For technical training programs addressing the AI skills gap, appropriate metrics might include time-to-proficiency for new skills, reduction in technical support escalations, or increased innovation outputs. Customer-facing training might measure improvements in satisfaction scores, reduced resolution times, or higher sales conversion rates following AI-driven learning interventions.
According to recent findings, teams using AI tools complete projects 33% faster and with 26% fewer resources, providing a benchmark for organizations to measure their own implementation success.
Techniques for Measuring ROI on AI Training Investments
Return on investment calculations for AI-powered training require sophisticated approaches that account for both direct cost impacts and value creation elements. The most effective ROI methodologies incorporate multiple calculation layers, beginning with efficiency gains in the training function itself.
Training production efficiency represents the most immediately measurable ROI component. Organizations using AI to create training materials typically report 40-70% reductions in content development time and associated costs. These efficiencies stem from automated content generation, translation services, customization capabilities, and reduced subject matter expert time requirements. For organizations with extensive training catalogs requiring frequent updates, these efficiency gains often justify AI investments independently of other benefits.
Learning acceleration delivers the second ROI layer through reduced time-to-competency. AI-powered adaptive learning typically reduces required training time by 20-50% compared to traditional approaches while maintaining or improving proficiency outcomes. This acceleration directly translates to productivity gains as employees become operational more quickly and require less time away from primary responsibilities for training activities.
Performance improvement constitutes the third and most valuable ROI component, though it requires the most sophisticated measurement approaches. Organizations demonstrating the highest returns employ techniques like controlled group comparisons, time-series analyses of performance data, and multi-variable regression models to isolate the impact of AI-enhanced training on specific business metrics. These approaches help distinguish training effects from other variables that might influence performance outcomes.
For organizations addressing the AI skills gap in technical roles, ROI calculations should incorporate opportunity cost metrics related to unfilled positions or project delays. The accelerated development of critical technical capabilities often delivers value far exceeding direct training cost reductions, particularly in competitive talent markets where external hiring proves challenging or expensive.
Continuous Improvement: Iterating on Feedback and Data
The distinguishing characteristic of high-performing AI training systems is their capacity for continuous, data-driven improvement. Unlike traditional training approaches that remain static between formal review cycles, AI-enhanced learning ecosystems can evolve constantly based on performance data and learner interactions.
Effective continuous improvement frameworks operate across multiple timescales simultaneously. Real-time adaptation occurs within individual learning sessions as AI systems adjust content difficulty, pacing, and examples based on learner responses and comprehension signals. This immediate responsiveness ensures learners remain appropriately challenged without experiencing frustration or disengagement.
Short-cycle improvements occur weekly or monthly as aggregate performance data reveals patterns across learner cohorts. These insights might trigger refinements to learning pathways, content sequencing, or assessment approaches. Organizations effectively leveraging these short-cycle improvements typically establish cross-functional review teams that include learning designers, data analysts, and business stakeholders who collaboratively interpret emerging patterns and authorize system adjustments.
Longitudinal improvements focus on strategic alignment and evolving business needs. These reviews examine longer-term trends in performance outcomes, emerging skill requirements, and shifting business priorities. Organizations leading in this area maintain dynamic competency frameworks that continuously evolve based on both internal performance data and external market intelligence about emerging skills.
The most sophisticated organizations implement bi-directional feedback loops between their AI training systems and workforce planning functions. Training engagement and performance data proactively inform talent acquisition strategies, role design decisions, and organizational structure planning. This integration ensures that insights about the AI skills gap gained through learning analytics directly influence broader talent management approaches.
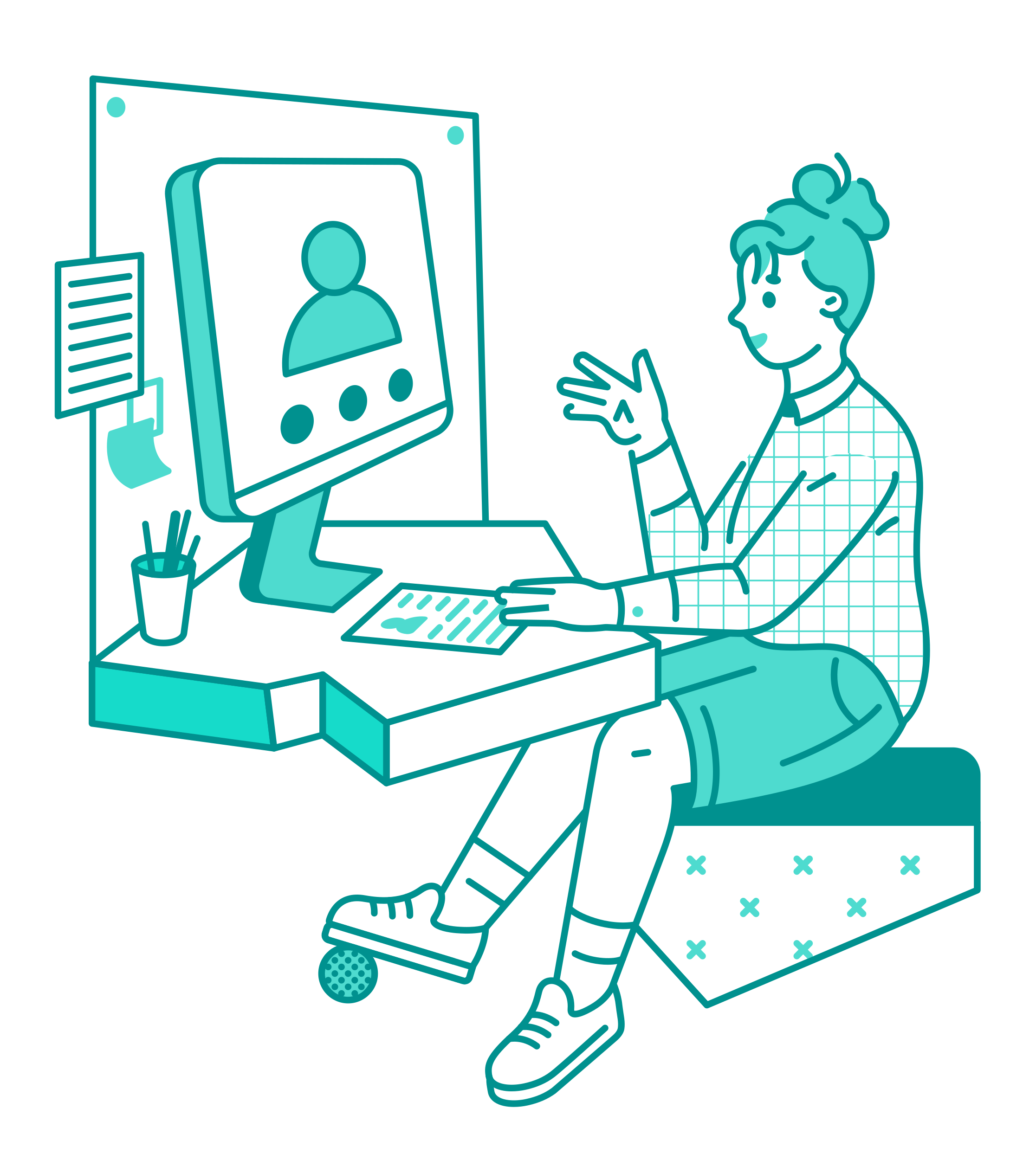
Ethics, Compliance, and the Future of AI in Employee Training
As artificial intelligence learning and development solutions become increasingly sophisticated and widespread, organizations must navigate complex ethical considerations and regulatory frameworks. The responsible implementation of these technologies requires balancing innovation with ethical standards and compliance requirements—a challenge that will only intensify as AI capabilities expand and regulations evolve.
Navigating Privacy and Ethical Concerns
The integration of AI employee training systems introduces significant privacy considerations that extend beyond traditional learning management approaches. These systems typically collect vast amounts of data including learning behaviors, performance patterns, content interactions, and sometimes even biometric responses such as eye tracking or facial expressions to gauge engagement and comprehension.
Organizations implementing AI corporate training must carefully consider both the explicit and implicit privacy implications of these data collection practices. Transparent communication with employees about what data is being collected, how it's being used, and the safeguards in place to protect sensitive information is essential not only for compliance but for maintaining trust. Leading organizations implement tiered consent models that allow employees to opt in or out of specific data collection practices based on their personal comfort levels.
Algorithmic bias represents another critical ethical concern requiring proactive management. AI systems trained on historical data risk perpetuating existing inequities in training opportunities and career advancement. For example, if historical data shows certain demographics received less advanced technical training in the past, AI recommendation engines might continue this pattern without appropriate intervention. Organizations must implement regular bias auditing processes that examine training recommendations, completion rates, and advancement opportunities across different demographic groups to identify and address potential disparities.
As Rumman Chowdhury eloquently states: "Responsible AI is not just about liability — it's about ensuring what you are building is enabling human flourishing." This principle should guide all ethical considerations in AI training implementation.
Ensuring Compliance with AI Legislation (e.g., EU AI Act)
The regulatory landscape governing AI applications in workplace learning is evolving rapidly, with the EU AI Act representing the most comprehensive framework to date. This legislation, effective February 2025, establishes specific requirements for AI systems used in employment contexts, including training and development applications.
Under the EU AI Act, AI employee training systems fall into different risk categories based on their specific functionality and potential impact on workers. Systems that merely deliver content or track basic completion metrics typically qualify as minimal risk applications. However, systems that make or influence decisions about career advancement, compensation, or continued employment face more stringent requirements as high-risk applications—requiring robust documentation, human oversight, and regular auditing.
A core compliance requirement under the Act is mandatory AI literacy training for all employees interacting with AI systems. This represents a significant shift in how organizations approach artificial intelligence learning and development—requiring not just the implementation of AI tools but also comprehensive education about how these systems work, their limitations, and appropriate use cases. Organizations must document this training and ensure it addresses both technical fundamentals and ethical considerations relevant to each employee's role.
Beyond European regulations, organizations must navigate a growing patchwork of regional and national AI governance frameworks. In the United States, while comprehensive federal legislation remains pending, states like California, Colorado, and New York have implemented specific requirements for algorithmic decision-making systems that affect employment. Similarly, regions including Canada, Singapore, and Australia have established AI ethics frameworks with specific provisions applicable to workplace implementations.
Practical compliance strategies include implementing dedicated AI governance committees with cross-functional representation from legal, HR, IT, and learning & development teams. These committees should establish clear protocols for evaluating new AI training technologies against relevant regulatory requirements, documenting design decisions that impact compliance, and maintaining auditable records of system performance and human oversight activities.

The Future Outlook: Emerging Trends and Innovations in AI Training
The evolution of AI employee training technologies continues to accelerate, with several transformative trends poised to reshape corporate learning in the coming years. Organizations that proactively anticipate these developments will be better positioned to capitalize on emerging opportunities while managing associated risks.
Recent data shows that 83% of companies now say AI is a top priority in their business plans (up from 67% in 2023), and 71% of C-suite executives identify AI training as essential for maintaining competitive advantage—underscoring the strategic importance of these capabilities.
Hyper-personalization represents perhaps the most significant near-term advancement, moving beyond basic adaptive content to truly individualized learning experiences. Next-generation AI corporate training platforms will incorporate multimodal learning style analysis—examining not just performance data but also preferred content formats, optimal learning times, attention patterns, and even cognitive load indicators to deliver precisely calibrated experiences. Research suggests these hyper-personalized approaches could improve skill acquisition rates significantly compared to current adaptive learning systems.
Virtual mentoring and AI coaching capabilities are rapidly maturing, shifting from scripted interactions to genuinely conversational guidance. These systems leverage advances in large language models and emotional intelligence algorithms to provide contextually relevant feedback, challenge assumptions, and scaffold complex skill development. While not replacing human mentorship, these AI coaches enable consistent, on-demand guidance at scale—particularly valuable for distributed workforces or specialized domains where expert mentors are scarce.
Dr. Matt Wood, VP Artificial Intelligence at AWS, notes: "I have not seen this level of engagement and excitement from customers, probably since the very, very early days of cloud computing." This enthusiasm reflects the transformative potential these technologies hold for employee development.
Perhaps most transformative is the emergence of AI-powered skills intelligence platforms that transcend traditional competency frameworks. These systems continuously analyze internal performance data, external market signals, and emerging industry trends to identify skill adjacencies, development pathways, and future capability requirements. Rather than relying on static role descriptions, organizations can leverage these dynamic skill ontologies to create continuously evolving learning pathways aligned with both individual career aspirations and organizational capability needs.
As these technologies evolve, organizations must maintain rigorous ethical oversight and human governance of AI training systems. The most successful implementations will leverage AI capabilities to enhance human potential rather than replace human judgment—creating hybrid learning ecosystems where technology and human expertise complement each other. This balanced approach, combined with proactive regulatory compliance, will characterize organizations that extract maximum value from AI while maintaining trust and ethical integrity.
As Paul Daugherty, Chief Technology and Innovation Officer at Accenture, wisely observes: "The reality is that being unprepared is a choice. The benefits come when we see AI as a tool, not a terror, and bring it into our sales motions." This philosophy applies equally to training—organizations that thoughtfully embrace AI as a training tool rather than viewing it with suspicion will realize the greatest benefits while maintaining human connection at the heart of learning.
Answers your employees need, right when they need them
Meet Botable — the AI chatbot that handles everything from simple FAQs to complex, multi-step questions, so your team can focus on what matters. Built for HR, QA, and beyond.
Continue reading
Ready to see what Botable can do for you?
Book your demo now to see how Botable can transform your workplace.
Identify your unique challenges
Flexible pricing options
Easy integrations
Step-by-step implementation plan
Customize Botable for your workflow
Book a demo
.webp)