CAPA System AI: The Future of Corrective and Preventive Actions
Quality control isn't just a checkbox—it's a critical business function that can make or break an organization. At the heart of effective quality management lies the Corrective and Preventive Action (CAPA) system, a framework increasingly being transformed by artificial intelligence. This transformation promises incremental improvements and a complete revolution in how organizations approach compliance and quality management.
Understanding CAPA Systems and the Role of AI
A CAPA system forms the backbone of quality management in regulated environments, serving as a structured approach to identifying, addressing, and preventing quality issues. These systems aren't merely reactive tools but strategic frameworks that enable continuous improvement across organizational processes. An effective CAPA system encompasses methodical identification of deviations, thorough root cause analysis, implementation of corrective actions, and verification of results.
Traditional CAPA systems, while essential, often suffer from inherent limitations:
- Manual processes create bottlenecks in issue resolution
- Subjective evaluations lead to inconsistent outcomes
- Siloed information impedes comprehensive analyses
These challenges significantly impact an organization's ability to maintain compliance and quality standards, especially as operations scale and regulatory requirements evolve.
The integration of AI into CAPA systems addresses these fundamental challenges by introducing automated analysis capabilities, pattern recognition across vast datasets, and predictive insights. According to current industry data, AI-assisted diagnostics and automation can reduce operational expenses by 50% while enhancing outcomes by 40%, demonstrating strong ROI metrics when applied to quality management processes.
What makes AI particularly valuable in CAPA system enhancement is its ability to learn and adapt over time. As the AI processes more quality incidents and their resolutions, it develops increasingly sophisticated models for predicting issues, recommending solutions, and optimizing preventive measures. This continuous learning cycle transforms the CAPA system from a reactive tool into a proactive quality management asset.

Decision Support for CAPA Verification
The verification phase of any CAPA management system represents a critical juncture where organizations determine whether implemented corrective actions have effectively resolved identified issues. Traditionally characterized by manual reviews and subjective assessments, AI technologies are fundamentally transforming this verification landscape through sophisticated decision support mechanisms.
AI-powered verification tools within a CAPA management system apply advanced algorithms to objectively assess the effectiveness of implemented actions. These tools analyze post-implementation data against predefined success metrics, providing quantitative evidence of resolution rather than relying solely on human judgment.
Large Language Models (LLMs) and generative AI have emerged as particularly valuable in compliance verification, offering frameworks for systematic rule interpretation and application. These systems:
- Process unstructured data from multiple sources
- Create comprehensive verification assessments
- Handle ambiguities through logical reasoning
- Make nuanced verification decisions in complex regulatory environments
Risk-based approaches powered by predictive analytics further enhance CAPA verification by prioritizing activities based on potential impact. The FDA has recognized this potential by introducing Predetermined Change Control Plans (PCCPs) to ensure the safety and continuous improvement of AI-enabled systems in regulated industries.
Importantly, AI systems maintain detailed audit trails of verification activities, documenting not only outcomes but also decision rationales. This transparency addresses regulatory expectations for explainability, particularly in highly regulated industries like healthcare and pharmaceuticals.
AI Assessment of CAPA Records
CAPA management processes generate extensive documentation that requires meticulous review to ensure regulatory compliance and effectiveness. AI technologies are revolutionizing this assessment process through automated analysis capabilities that far exceed traditional manual reviews in both speed and accuracy.
AI-powered document assessment leverages natural language processing (NLP) to systematically evaluate CAPA records against established regulatory frameworks and internal standards. These systems can analyze thousands of documents in minutes, identifying:
- Gaps in documentation
- Inconsistencies in root cause analysis
- Inadequate corrective action plans
- Potential compliance issues before they become violations
By maintaining a continuously updated knowledge base of regulatory requirements, these AI systems ensure CAPA management documentation remains compliant even as regulations evolve. This is particularly valuable as the FDA introduces frameworks like PCCPs that focus on maintaining or improving safety while allowing real-time data updates.
Machine learning algorithms further enhance assessment capabilities by identifying patterns across CAPA records that might indicate systemic issues. By aggregating data from manufacturing logs, supplier records, customer complaints, and historical CAPA documentation, AI systems can detect recurring problems that might escape notice in isolated record reviews.
AI Prioritization of Quality Issues
In any quality management framework, not all issues carry equal weight or urgency. Modern CAPA management software enhanced with AI capabilities brings unprecedented precision to the prioritization process, moving beyond traditional risk matrices to deliver dynamic, data-driven prioritization.
Advanced CAPA management software now incorporates machine learning algorithms that continuously analyze incoming quality issues against multiple dimensions of risk and impact. These systems evaluate factors including:
- Potential patient or consumer harm
- Regulatory implications
- Production impacts
- Historical resolution patterns
Fuzzy Logic in Quality Assessment: Unlike traditional binary classification systems, fuzzy logic allows for nuanced evaluations that better reflect real-world complexities. This approach is especially effective when prioritizing issues that involve ambiguous risk factors or require subject matter expertise.
Natural Language Processing for Unstructured Data: Modern CAPA management software can analyze customer complaints, audit findings, and investigation reports to identify recurring themes and escalate issues that indicate potential systemic problems. This capability has been demonstrated in implementations like HEICO Companies' use of the PSI AI Advisor, which analyzed 14,360 records and identified 823 potential severe cases with 85%+ accuracy.
Dynamic Reprioritization: The most sophisticated CAPA management software implements capabilities that adjust issue rankings as new information becomes available. If additional complaints emerge about a specific product, or if similar issues are reported across multiple facilities, the system automatically elevates the priority level to reflect this increased risk profile.
Predictive Quality Assessment (PQA): These systems analyze historical data patterns to forecast potential quality issues before they materialize. Organizations utilizing PQA capabilities can address emerging issues at their earliest stages, often preventing them from developing into significant quality problems that would require formal CAPA processes.

The Integration of AI into CAPA Systems
The successful integration of AI into CAPA software requires a strategic, phased approach that balances technological innovation with practical implementation considerations. Organizations achieving the greatest success in this transformation begin with a comprehensive assessment of their current CAPA processes, identifying specific pain points and inefficiencies that AI can address.
Modern CAPA software enhanced with AI capabilities represents a significant evolution from traditional quality management tools. The integration process typically begins with pilot implementations focused on specific functions, such as automated data analysis or predictive risk assessment. This approach aligns with current industry trends, as McKinsey reports that 78% of companies are now utilizing AI (up from 20% in 2017), with measurable improvements in compliance and efficiency.
Data readiness presents a critical challenge when integrating AI into CAPA software. Organizations must ensure their quality data is sufficiently structured, cleaned, and contextualized to support effective AI algorithms. This often requires establishing robust data governance frameworks and investing in data preparation activities before full AI implementation.
Beyond technical considerations, workforce preparation represents a crucial success factor in AI integration. Organizations must address both skill development needs and potential resistance to change. Effective implementation strategies include:
- Creating AI champions within quality teams
- Providing comprehensive training on new CAPA software capabilities
- Clearly communicating how AI augments rather than replaces human expertise
The most sophisticated implementations establish continuous improvement mechanisms for the AI systems themselves, ensuring that capabilities evolve alongside the organization's quality management needs.
{{cta}}
Regulatory Perspectives on AI in CAPA Systems
Regulatory agencies have established increasingly detailed frameworks for AI implementation in quality management systems, including CAPA processes. These guidelines emphasize validation, documentation, and human oversight requirements.
FDA Guidance
The FDA has introduced a seven-step risk-based credibility assessment plan for AI-enabled systems. This plan helps evaluate AI models' outputs for specific contexts of use and covers data management, model design, training, validation, and performance monitoring.
For CAPA systems specifically, the FDA recommends detailed documentation in submissions, including:
- Information about AI model architecture
- Data collection and processing methods
- Device performance metrics
- How AI features support intended uses
- Risk management strategies
- Integration into clinical workflows or quality processes
Human Oversight Requirements
Regulatory authorities stress the necessity of human agency in AI-powered quality systems. Oversight models such as human-in-the-loop (HITL), human-on-the-loop (HOTL), and human-in-command (HIC) aim to ensure humans can monitor, intervene, or override AI systems during critical tasks, particularly in high-risk applications.
For CAPA processes specifically, the FDA encourages human oversight, treating systems with higher autonomy as riskier. These systems need more significant safeguards and human intervention to confirm outputs before implementation in quality decisions.

AI Impact on Quality and Compliance
Integrating artificial intelligence into quality management and compliance processes yields measurable improvements across multiple dimensions of organizational performance. Early adopters report significant reductions in quality-related costs, with some manufacturers documenting 30-40% decreases in expenses associated with defects, rework, and customer complaints.
Beyond cost savings, AI-powered quality systems are demonstrating remarkable improvements in compliance posture. Healthcare AI applications alone are projected to grow at a compound annual growth rate (CAGR) of 37% from 2023 to 2030, with substantial benefits tied to automation and predictive analytics in regulated processes.
Documentation represents another area where AI delivers substantial improvements. During audits, AI capabilities allow organizations to rapidly produce comprehensive evidence of compliance, reducing audit duration and associated stress. This is particularly valuable as regulatory frameworks like the FDA's PCCPs require detailed documentation of AI model architecture, data collection/processing, and device performance.
Perhaps most significantly, AI enables a shift from reactive to predictive quality management. Advanced predictive models can identify patterns in operational data that indicate emerging quality or compliance risks before they manifest as actual problems. This aligns with the FDA's emphasis on lifecycle maintenance plans to monitor AI system performance as new data or updates emerge.
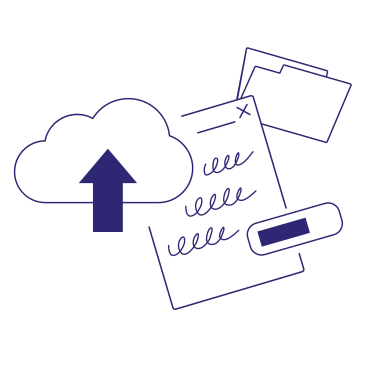
Getting Started with AI-Powered CAPA Implementation
Implementing AI in your CAPA system requires a structured approach. Here's a practical framework to guide your journey:
1. Assessment and Planning
- System Evaluation: Audit your current CAPA processes to identify bottlenecks, inefficiencies, and areas where AI could provide the most value
- Data Readiness Assessment: Evaluate the quality, accessibility, and structure of your data
- Stakeholder Mapping: Identify key personnel across quality, IT, regulatory, and operations departments who should be involved
2. Foundation Building
- Data Preparation: Clean, structure, and organize quality data to ensure it's AI-ready
- Infrastructure Development: Establish the necessary technical infrastructure (cloud resources, integration points, security measures)
- Regulatory Alignment: Review current guidelines from regulatory bodies relevant to your industry
3. Pilot Implementation
- Select a Focused Use Case: Begin with a single high-value application (e.g., complaint categorization, root cause analysis)
- Develop and Test: Build an initial AI model focused on your selected use case
- Measure Results: Establish clear metrics to evaluate the AI's performance against current processes
4. Validation and Expansion
- Formal Validation: Conduct thorough validation following industry standards
- Training and Change Management: Prepare your team for broader AI implementation
- Incremental Expansion: Gradually extend AI capabilities to additional CAPA processes
5. Continuous Improvement
- Performance Monitoring: Implement dashboards to track AI effectiveness
- Regular Updates: Schedule periodic reviews of AI models to ensure they remain current
- Regulatory Scanning: Monitor for regulatory changes that may impact AI usage in quality systems
AI Terminology Explained
Natural Language Processing (NLP): Technology that enables computers to understand, interpret, and generate human language. In CAPA systems, NLP helps analyze unstructured text in complaints, audit findings, and investigation reports.
Machine Learning: A subset of AI that allows systems to automatically learn and improve from experience without being explicitly programmed. In CAPA contexts, machine learning algorithms can identify patterns in quality data to predict potential issues.
Fuzzy Logic: A mathematical approach that allows for degrees of truth rather than the binary true/false values of conventional logic. This is valuable in CAPA prioritization where issues may not fit neatly into predefined categories.
Predictive Analytics: Using data, statistical algorithms, and machine learning techniques to identify the likelihood of future outcomes based on historical data. CAPA systems use this to forecast potential quality issues before they occur.
Human-in-the-Loop (HITL): An approach where human judgment is incorporated into AI system operations at critical decision points. Regulatory bodies often require HITL in high-risk quality management applications.
Answers your employees need, right when they need them
Meet Botable — the AI chatbot that handles everything from simple FAQs to complex, multi-step questions, so your team can focus on what matters. Built for HR, QA, and beyond.
Continue reading
Ready to see what Botable can do for you?
Book your demo now to see how Botable can transform your workplace.
Identify your unique challenges
Flexible pricing options
Easy integrations
Step-by-step implementation plan
Customize Botable for your workflow
Book a demo
.webp)