The 5 Whys Method Using AI - Uncover Real Solutions Fast
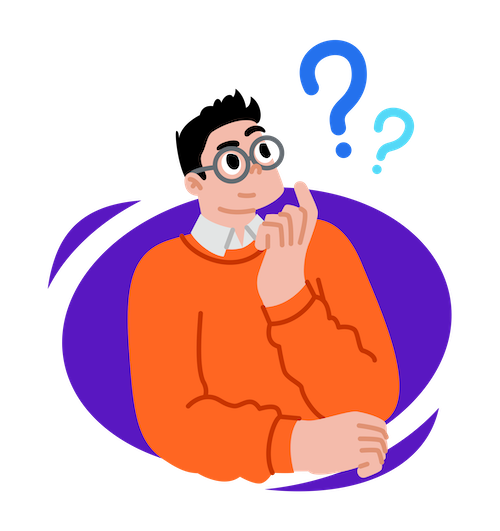
Imagine a straightforward yet effective technique that swiftly identifies the root cause of any issue—introducing the 5 Whys method, now enhanced by artificial intelligence. This innovative fusion of human intuition and machine learning is revolutionizing how we tackle complex problems, offering deeper insights and more effective solutions than ever before. In this article, we'll explore how the 5 Whys technique, when supercharged by AI, can transform your problem-solving prowess and lead you to breakthrough solutions that stick.
Introduction to the 5 Whys Method
Defining the 5 Whys: A Root Cause Analysis Tool
The 5 Whys technique is a powerful root cause analysis tool that has been helping businesses and individuals solve problems for decades.
What is the 5 Whys Process?
When faced with a problem, you ask "why" five times to drill down to the root cause. This iterative questioning peels back the layers of symptoms to reveal the underlying issue that needs addressing.
The beauty of the 5 Whys framework lies in its versatility and simplicity. It can be applied to a wide range of scenarios, from manufacturing defects to customer service issues, and even personal challenges. By repeatedly asking "why," you're forced to look beyond the surface-level problem and dig deeper into the causal factors that contribute to the issue at hand.
What sets the 5 Whys tool apart from other problem-solving methods is its focus on depth rather than breadth. Instead of brainstorming multiple possible causes, it encourages a linear, focused approach that often leads to surprising revelations about the true nature of the problem. This targeted inquiry helps teams avoid the common pitfall of addressing symptoms rather than causes, leading to more effective and lasting solutions.
Brief History and Origin of the 5 Whys Technique
The 5 Whys technique has its roots in the Toyota Production System, a manufacturing methodology developed by the Japanese automaker in the mid-20th century. Sakichi Toyoda, the founder of Toyota Industries, is credited with creating this approach as part of the company's relentless pursuit of continuous improvement, known as "Kaizen" in Japanese.
Toyoda recognized that to truly solve problems and prevent their recurrence, it was essential to identify and address the root cause. He observed that by asking "why" multiple times, his team could peel back the layers of an issue and uncover the fundamental reason behind a problem. This simple yet effective method quickly became an integral part of Toyota's problem-solving culture.
The success of the 5 Whys analysis at Toyota didn't go unnoticed. As the company's reputation for quality and efficiency grew, so did interest in its management practices. In the 1970s and 1980s, as Western manufacturers sought to emulate Toyota's success, the 5 Whys technique gained popularity beyond Japan's borders. It became a cornerstone of lean manufacturing principles and Six Sigma methodologies, which aim to reduce waste and improve quality in various industries.
Over time, the 5 Whys framework has evolved and been adapted for use in fields far beyond manufacturing. Today, it's employed in software development, healthcare, education, and even personal development. Its enduring popularity is a testament to its effectiveness and adaptability in an ever-changing business landscape.
The 5 Whys technique is once again evolving. By combining this time-tested method with the power of AI, we're entering a new frontier of problem-solving capabilities. This synergy between human intuition and machine learning promises to enhance our ability to uncover root causes and develop innovative solutions to complex challenges.

The Synergy Between the 5 Whys Method and AI
Enhancing Problem-Solving with AI-Powered Tools
The integration of artificial intelligence with the 5 Whys technique marks a significant leap forward in problem-solving methodologies. This powerful combination enhances our ability to uncover root causes and develop effective solutions in ways that were previously unimaginable. By leveraging AI's data processing capabilities and pattern recognition, we can now apply the 5 Whys in problem solving with unprecedented depth and accuracy.
One of the most exciting applications of this synergy is in the realm of AI projects themselves. In AI projects, the 5 Whys helps in debugging the entire pipeline from data collection to model evaluation and ensures transparency in AI decision-making processes. This recursive approach allows developers and data scientists to identify and address issues at every stage of the AI lifecycle, leading to more robust and reliable systems.
AI-powered tools can significantly enhance the 5 Whys process by:
- Data Analysis: AI can quickly sift through vast amounts of data to identify patterns and correlations that might not be immediately apparent to human analysts.
- Natural Language Processing: Advanced NLP algorithms can analyze text-based feedback, customer complaints, or incident reports to extract key themes and potential root causes.
- Predictive Analytics: AI models can forecast potential issues before they occur, allowing for proactive application of the 5 Whys technique.
- Visualization: AI-generated visualizations can help teams better understand complex cause-and-effect relationships uncovered during the 5 Whys process.
Perhaps one of the most valuable aspects of this synergy is its impact on organizational culture. The technique promotes continuous improvement and fosters a culture of transparency and trust by providing clear explanations of AI's decision-making processes. This transparency is crucial in building confidence in AI systems and ensuring that the insights generated are actionable and understood by all stakeholders.
By combining the structured inquiry of the 5 Whys with the analytical power of AI, organizations can develop a more nuanced understanding of their challenges. This enhanced problem-solving capability leads to more targeted interventions, reduced downtime, and improved overall efficiency. As AI continues to evolve, we can expect even more sophisticated applications of the 5 Whys technique, further revolutionizing how we approach and solve complex problems across various industries.
Step-by-Step Guide: 5 Whys Method Using AI Chatbots
Assembling a Multidisciplinary Team for Diverse Insights
The first step in implementing the 5 why process with AI assistance is to assemble a diverse team. This multidisciplinary approach ensures that you have a range of perspectives and expertise to tackle the problem at hand. Include members from different departments, levels of seniority, and areas of expertise. AI can assist in this process by analyzing team composition and suggesting additional members based on the specific problem and historical data of successful problem-solving teams.
Clearly Defining the Problem at Hand
Before diving into the 5 why methodology, it's crucial to clearly define the problem. AI tools can help by analyzing data from various sources, such as customer complaints, production logs, or employee feedback, to identify recurring issues or patterns. This data-driven approach ensures that you're addressing the most pressing problems and not just symptoms of larger issues.
The Iterative Process of Asking "Why"
The core of the 5 whys root cause analysis lies in the iterative questioning process. Here's how AI can enhance this step:
- Initial "Why": AI analyzes the problem statement and suggests potential first-level causes based on historical data and industry trends.
- Subsequent "Whys": For each answer, the AI proposes follow-up questions, considering the context and previous responses.
- Data Validation: As you progress through the "whys," AI cross-references your answers with available data to ensure accuracy and relevance.
- Branching Possibilities: If multiple root causes are possible, AI can help you explore different branches simultaneously, providing a more comprehensive analysis.
- Stopping Criteria: AI assists in determining when you've reached a true root cause, based on predefined criteria and patterns from successful past analyses.

Finding and Acting on the Root Cause
Once you've completed the 5 whys analysis example, AI can help synthesize the information and identify the most likely root cause. It can also suggest potential solutions based on similar problems solved in the past or best practices in your industry. The AI might present a 5 why analysis example from a similar situation to guide your decision-making process.
Monitoring Changes and Documenting Results
After implementing solutions, it's crucial to monitor their effectiveness. AI can:
- Track key performance indicators related to the identified problem.
- Analyze data in real-time to detect any recurrence of the issue.
- Generate comprehensive reports documenting the entire 5 why process, from problem identification to solution implementation and results.
- Suggest refinements to the solution based on ongoing performance data.
By following this AI-assisted 5 why process, you can uncover root causes more efficiently and effectively than ever before. The combination of human insight and AI capabilities ensures a thorough, data-driven approach to problem-solving that can be consistently applied across your organization.
Key Considerations and Best Practices When Using the 5 Whys Method with AI
Avoiding Common Pitfalls and Ensuring Accurate Cause Identification
When implementing 5 whys problem-solving with AI assistance, it's crucial to be aware of potential pitfalls that could lead to inaccurate conclusions. One common issue is confirmation bias, where teams may unconsciously steer the analysis towards preconceived notions. AI can help mitigate this by providing objective data-driven insights, but it's essential to regularly challenge and validate AI-generated suggestions.
Another pitfall is the tendency to stop at surface-level causes. AI can assist by prompting deeper questioning and highlighting when responses are too shallow or generic. However, human oversight is crucial to ensure that the AI doesn't miss context-specific nuances that might not be apparent in the data.
To ensure accurate cause identification:
- Regularly calibrate the AI model with updated data and feedback from successful problem-solving sessions.
- Implement a system of checks and balances where human experts review and validate AI-generated insights.
- Encourage team members to question AI suggestions and provide alternative perspectives.
The Role of Data in Enhancing Root Cause Analysis
Data plays a pivotal role in enhancing the effectiveness of 5 whys problem-solving when combined with AI. High-quality, relevant data can significantly improve the accuracy and depth of the analysis. Here's how to leverage data effectively:
- Data Collection: Implement robust systems to collect relevant data across all aspects of your operations. This could include production metrics, customer feedback, employee surveys, and more.
- Data Integration: Ensure that your AI system can access and analyze data from various sources to provide a comprehensive view of the problem.
- Historical Analysis: Use historical data to identify patterns and trends that may not be immediately apparent, helping to uncover deeper root causes.
- Real-time Data Processing: Implement AI systems capable of processing real-time data to provide up-to-date insights during the 5 whys process.
- Data Visualization: Utilize AI-powered visualization tools to present complex data in easily understandable formats, aiding in the identification of root causes.
Balancing Human Insight with AI Capabilities
While AI can significantly enhance the 5 whys problem-solving process, it's crucial to strike the right balance between AI capabilities and human insight. Here are some strategies to achieve this balance:
Collaborative Approach: Encourage a collaborative environment where team members work alongside AI tools, using the technology to augment their problem-solving skills rather than replace them.
Critical Thinking: Train team members to evaluate AI-generated insights critically, understanding the technology's strengths and limitations.
Contextual Understanding: Recognize that humans often have a deeper understanding of context and nuanced factors that may not be captured in data. Encourage team members to contribute this contextual knowledge to enrich the AI-driven analysis.
Continuous Learning: Implement a feedback loop where human insights are used to improve and refine AI models over time, creating a symbiotic relationship between human and artificial intelligence.
Ethical Considerations: Be mindful of potential biases in AI systems and ensure that ethical considerations are always at the forefront of decision-making processes.
By carefully considering these factors and implementing best practices, organizations can harness the full potential of AI-assisted 5 whys problem-solving while avoiding common pitfalls. This balanced approach leads to more accurate root cause identification and more effective problem resolution.
{{cta}}
Limitations and Challenges of Root Cause Analysis Using AI
Understanding the Limitations of AI in Identifying Root Causes
While AI has significantly enhanced our ability to perform root cause analysis, it's crucial to understand its limitations. One of the primary challenges is the potential for AI to miss context-specific nuances that human experts might catch. For instance, in a 5 whys root cause analysis example, an AI might not fully grasp the intricacies of human behavior or organizational culture that could be contributing factors to a problem.
Critics suggest that the 5 Whys method can be too simplistic and may not always uncover multiple root causes or probe deeper than the client's level of knowledge. This limitation can be exacerbated when relying solely on AI, as the system's analysis is only as good as the data it's trained on. If the training data doesn't include a wide range of 5 why examples for root cause scenarios, the AI might struggle with novel or complex situations.
Another limitation is the potential for AI to perpetuate biases present in historical data. If past problem-solving efforts were influenced by biased decision-making, an AI system might inadvertently reinforce these biases in its analysis and recommendations.
Navigating Challenges: When to Trust AI Over Human Judgement
Determining when to trust AI over human judgment in root cause analysis is a delicate balance. AI excels in processing vast amounts of data and identifying patterns that might be invisible to human analysts. In scenarios where objective data analysis is crucial, such as in manufacturing defects or software bugs, AI-driven insights can be particularly valuable.
However, human judgment becomes indispensable when dealing with:
- Novel situations with little historical data
- Problems involving complex human interactions or emotions
- Scenarios requiring ethical considerations or value judgments
A hybrid approach, where AI suggestions are critically evaluated by human experts, often yields the best results. This approach allows for the leveraging of AI's data processing capabilities while incorporating human intuition and contextual understanding.
Emerging Trends and Future Developments in AI-Assisted Problem Solving
The future of AI-assisted problem solving, particularly in the context of the 5 Whys method, is promising. Here are some emerging trends and potential developments:
Advanced Natural Language Processing: Future AI systems may be able to conduct more nuanced conversations during the 5 Whys process, asking follow-up questions and probing deeper based on contextual understanding.
Multi-modal Analysis: AI could integrate data from various sources (text, audio, video, sensor data) to provide a more comprehensive root cause analysis.
Explainable AI: As AI systems become more complex, there's a growing emphasis on making their decision-making processes transparent and explainable, which is crucial for building trust in AI-assisted problem-solving.
Real-time Adaptive Learning: Future AI systems might be able to learn and adapt their analysis in real-time based on new information or feedback, making the 5 Whys process more dynamic and responsive.
Integration with IoT and Big Data: As more devices become connected, AI-assisted root cause analysis could tap into vast networks of real-time data, providing unprecedented insights into complex systems and processes.
Predictive Root Cause Analysis: AI might evolve to not only identify current root causes but also predict potential future issues based on emerging patterns and trends.
As these technologies develop, we'll likely see more sophisticated 5 Whys root cause analysis examples that combine the strengths of AI and human expertise. The key to successful implementation will be maintaining a balance between leveraging advanced AI capabilities and preserving the critical role of human insight and judgment in the problem-solving process.
Tagged:
Thinking about Botable for your team? See it live
.png)
In just 30 minutes, see how Botable can transform your workplace with a personalized demo.
Identify your unique challenges
Flexible pricing options
Easy integrations
Step-by-step implementation plan
Customize Botable for your workflow
Meet with the team



.png)