Boost Efficiency: Chatbots & Continuous Feedback Loops in Your QMS

Businesses constantly seek innovative ways to enhance employee experience and streamline operations. Enter chatbots: the tireless digital assistants revolutionizing how companies interact with employees. But what separates a good chatbot from a great one? The answer lies in the power of quality improvement feedback loops using chatbots. This article delves into how these feedback mechanisms are enhancing chatbot performance and fundamentally reshaping the landscape of AI-driven employee service.
Introduction to Chatbots and Continuous Improvement Feedback Loops
In recent years, chatbots have evolved from simple rule-based programs to sophisticated AI-powered assistants capable of handling complex queries and tasks. This evolution, however, didn't happen in isolation. It results from a critical process called continuous improvement, fueled by feedback loops.
The vital role of feedback in evolving chatbot technologies
Feedback is the lifeblood of chatbot evolution, helping to identify and correct mistakes that might otherwise hinder development. Without it, these AI assistants would remain static, unable to adapt to changing user needs or correct their shortcomings. The importance of feedback in chatbot development cannot be overstated. It is a compass guiding developers and AI models towards more accurate, efficient, and user-friendly interactions.
Feedback loops allow AI chatbots to learn and improve their accuracy over time, leading to significant improvements across all metrics. This continuous learning process sets advanced chatbots apart from their more basic counterparts. Chatbots can dramatically enhance their performance and satisfaction by analyzing user interactions, identifying pain points, and adapting responses.
Understanding continuous improvement in the context of AI and chatbots
Continuous improvement in AI and chatbots is not a one-time fix but an ongoing process. It involves constantly gathering data, analyzing performance, and making incremental changes to enhance the chatbot's capabilities. This iterative approach allows for rapid adaptation to user needs and preferences, ensuring the chatbot remains relevant and practical.
In the context of AI chatbots, continuous improvement manifests in several ways:
- Enhanced Natural Language Processing (NLP): As chatbots interact with more users, they can refine their language understanding, leading to more accurate interpretations of user queries.
- Expanded knowledge base: Feedback loops help identify gaps in the chatbot's knowledge, allowing developers to add new information and capabilities.
- Improved conversation flow: By analyzing user interactions, chatbots can learn to navigate conversations more smoothly, reducing frustration and improving user experience.
- Personalization: Over time, chatbots can learn to tailor their responses based on individual user preferences and behavior patterns.
The synergy between chatbots and continuous improvement feedback loops creates a powerful business tool. As these AI assistants become more adept at understanding and responding to user needs, they boost efficiency and elevate the overall employee experience. In the following sections, we'll explore how to craft effective feedback loops, implement enhancement strategies, and leverage these insights for competitive advantage in the ever-evolving landscape of AI-driven employee service.

Crafting Effective Feedback Loops for Chatbots
Implementing well-designed feedback loops—often referred to as machine learning feedback loops or AI feedback loops—is crucial to maximizing chatbots' potential. These loops are the backbone of continuous improvement in chatbot technology. By integrating quality improvement feedback loops using chatbots into Quality Management Systems (QMS), businesses can ensure their chatbots are constantly evolving to meet user needs and expectations.
Essential elements of a chatbot feedback loop
A robust chatbot feedback loop comprises several key components that drive continuous improvement. These elements form the foundation of QMS feedback loops, ensuring the chatbot's performance is consistently monitored, analyzed, and enhanced.
Identifying feedback sources: Employees, analytics, and internal teams
Chatbot feedback can be collected from in-conversation feedback and external sources, such as support emails, usability testing, and social channels[3][4]. This multi-faceted approach to gathering feedback ensures a comprehensive understanding of the chatbot's performance and user satisfaction.
- User feedback: Direct input from users interacting with the chatbot.
- Analytics data: Metrics on usage patterns, conversation flows, and user engagement.
- Internal team insights: Observations and suggestions from employee service representatives and developers.
By tapping into these diverse sources, businesses can gain a holistic view of their chatbot's strengths and areas for improvement.
{{cta}}
Categorizing feedback: Enhancing accuracy and user experience
Once feedback is collected, it's essential to categorize it effectively. This process helps prioritize improvements and address specific aspects of the chatbot's performance. Common categories include:
- Accuracy of responses
- Natural language understanding
- Conversation flow
- User interface and experience
- Technical issues or bugs
Categorizing feedback in this manner allows for targeted improvements and helps maintain a structured approach to chatbot enhancement within the QMS framework.
Techniques to gather actionable feedback
Gathering meaningful feedback is crucial for the success of AI feedback loops. Here are some effective techniques to collect actionable insights:
Direct user surveys and indirect insights (Usage patterns, support inquiries)
- In-chat surveys: Short, non-intrusive questions to gauge user satisfaction at the end of conversations.
- Follow-up emails: Detailed surveys are sent to users after interacting with the chatbot.
- Usage pattern analysis: Examining how users navigate conversations and where they might struggle.
- Support ticket analysis: Review cases where users needed additional human support after chatbot interactions.
These methods provide quantitative and qualitative data, offering a comprehensive view of the chatbot's performance and user experience.
Leveraging machine learning for feedback analysis
Machine learning plays a crucial role in analyzing the vast feedback data collected. By implementing machine learning feedback loops, businesses can:
- Identify patterns and trends in user behavior and satisfaction levels.
- Automatically categorize and prioritize feedback based on urgency and impact.
- Predict potential issues before they become widespread problems.
- Generate actionable insights from unstructured feedback data, such as free-text comments.
By integrating these techniques into QMS feedback loops, organizations can create a systematic approach to chatbot improvement. This ensures that the AI-driven employee service solution remains aligned with quality standards and continuously evolves to meet user needs.
Crafting effective quality improvement feedback loops using chatbots is an ongoing process that requires dedication and a willingness to adapt. By leveraging diverse feedback sources, categorizing insights effectively, and utilizing advanced analysis techniques, businesses can integrate knowledge management to create chatbots that meet current needs and anticipate future user expectations.
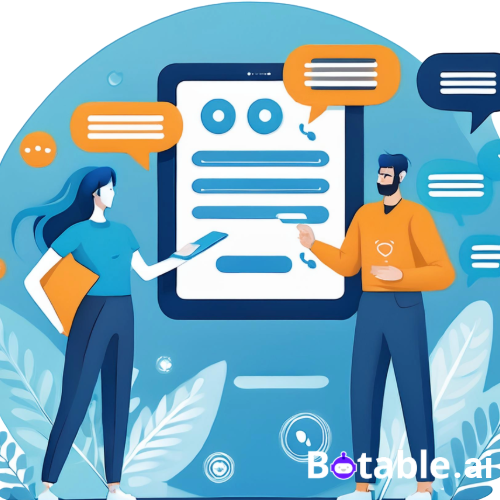
Turning Feedback into Chatbot Enhancement Strategies
Transforming raw feedback into tangible improvements is crucial for the evolution of chatbot systems. This transformation is at the heart of artificial intelligence feedback loops, enabling chatbots to adapt and grow based on real-world interactions and user needs.
Incorporating feedback: Manual vs semi-automated updates
When implementing changes based on feedback, organizations typically choose between manual and semi-automated approaches. Each method has its merits and challenges, and the choice often depends on the complexity of the chatbot system and the resources available.
The role of human oversight in refining AI models
Human oversight remains critical in refining AI models, even in highly automated systems. Expert human analysts play several vital roles:
- Interpreting complex feedback that may be beyond the current capabilities of AI systems
- Identifying nuanced patterns or cultural contexts that machines might miss
- Making strategic decisions about which improvements to prioritize
- Ensuring ethical considerations are maintained throughout the enhancement process
Analyzing feedback data helps prioritize fixes and improvements for chatbot models, ensuring they meet evolving employee expectations. This human-led analysis is crucial for maintaining the quality and relevance of chatbot interactions.
Semi-automated methods: Retraining models with new data sets
Semi-automated methods leverage the power of artificial intelligence feedback systems while still maintaining a degree of human control. This approach typically involves:
- Automated collection and initial processing of feedback data
- AI-driven analysis to identify trends and potential areas for improvement
- Human review of AI-generated insights and recommendations
- Supervised retraining of chatbot models using curated datasets
This hybrid approach allows for rapid iteration and improvement while ensuring that changes align with business goals and maintain high-quality standards.
Critical areas for improvement based on feedback
Feedback often highlights mistakes and areas where chatbots can be enhanced to provide better user experiences and more efficient interactions.
Navigational flows and user engagement tactics
Improving navigational flows is crucial for maintaining user engagement and reducing frustration. Key strategies include:
- Simplifying conversation paths to help users reach their goals more quickly
- Implementing more intuitive menu structures and quick reply options
- Enhancing the chatbot's ability to guide users back on track when conversations veer off course
- Incorporating personalized recommendations based on user history and preferences
By focusing on these aspects, chatbots can provide a more seamless and engaging experience, encouraging users to rely on them for future interactions.
Expanding intent coverage and reducing misunderstanding rates
One of the most critical areas for improvement in chatbot systems is enhancing their ability to accurately understand and respond to user intents. Strategies for this include:
- Broadening the range of intents, the chatbot can recognize and respond to
- Improving natural language processing capabilities to handle variations in user input better
- Implementing more sophisticated disambiguation techniques to clarify user intentions
- Developing better fallback mechanisms for when the chatbot encounters unfamiliar queries
By continuously expanding intent coverage and reducing misunderstandings, chatbots can effectively handle a wider range of user queries, leading to higher satisfaction rates and reduced need for human intervention.
Turning feedback into actionable enhancement strategies is an ongoing process that requires a balance of human insight and artificial intelligence capabilities. By leveraging manual oversight and semi-automated methods, organizations can ensure their chatbots evolve in line with user needs and expectations. Focusing on key areas such as navigational flows and intent coverage allows for targeted improvements that significantly enhance the chatbot experience.
Monitoring the Success and Evolution of Your Chatbot's Feedback Loop
To ensure the continuous improvement of your chatbot, it's crucial to implement a robust monitoring system that tracks the effectiveness of your feedback loop. This process involves establishing clear metrics, regularly assessing performance, and making data-driven adjustments to your strategy.
Establishing metrics for success: User satisfaction, resolution rate, continuous engagement metrics
When evaluating the success of your chatbot's feedback loop, it's essential to focus on key performance indicators (KPIs) that reflect both user experience and operational efficiency. Some critical metrics to consider include:
- User Satisfaction: Measure through post-interaction surveys or sentiment analysis.
- Resolution Rate: The percentage of queries successfully resolved by the chatbot without human intervention.
- Continuous Engagement: Track repeat usage and the depth of user interactions over time.
- Response Accuracy: Monitor the chatbot's ability to provide correct and relevant information.
- Conversation Duration: Assess the efficiency of interactions by measuring average conversation length.
Plotting bot success metrics before and after implementing feedback loops helps assess the effectiveness of the loops in enhancing chatbot performance. This before-and-after comparison provides tangible evidence of improvement and helps identify areas requiring further attention.
Adjusting your strategy based on performance data
As you gather data on your chatbot's performance, it's crucial to use these insights to refine and optimize your strategy continually.
Regular assessments: What's working and what needs tweaking?
Conduct regular performance reviews to identify strengths and weaknesses in your chatbot's functionality. Consider the following approaches:
- Analyze user feedback trends to spot recurring issues or praise.
- Review conversation logs to identify common stumbling points or mistakes, as well as successful interaction patterns.
- Examine resolution rates across different types of queries to pinpoint areas for improvement.
- Measuring intent coverage over time indicates whether the feedback loop increases the chatbot's ability to map user messages to the correct intent. This metric is crucial for understanding the chatbot's learning progress.
By systematically evaluating these aspects, including implementing quality improvement feedback loops using chatbots, you can make informed decisions about where to focus your improvement efforts.
The importance of maintaining a dynamic improvement process
The landscape of user needs and technological capabilities is constantly evolving. To keep your chatbot relevant and effective, it's essential to maintain a dynamic improvement process that can adapt to these changes. Consider the following strategies:
- Implement an agile development approach that allows for rapid iterations and updates.
- Regularly review and update your success metrics to ensure they align with current business goals and user expectations.
- Foster a culture of continuous learning within your team, encouraging ongoing exploration of new technologies, knowledge management, and best practices in chatbot development.
- Establish a feedback mechanism for your improvement process, allowing team members to suggest enhancements to how you gather and implement feedback.
Maintaining a flexible and responsive approach to chatbot improvement ensures that your AI assistant remains at the forefront of employee service technology, consistently meeting and exceeding user expectations.
Monitoring the success and evolution of your chatbot's feedback loop is not a one-time task but an ongoing commitment to excellence. By establishing clear metrics, regularly assessing performance, and maintaining a dynamic improvement process, you can create a chatbot that meets current needs and adapts to future challenges and opportunities in the ever-changing landscape of AI-driven employee service.
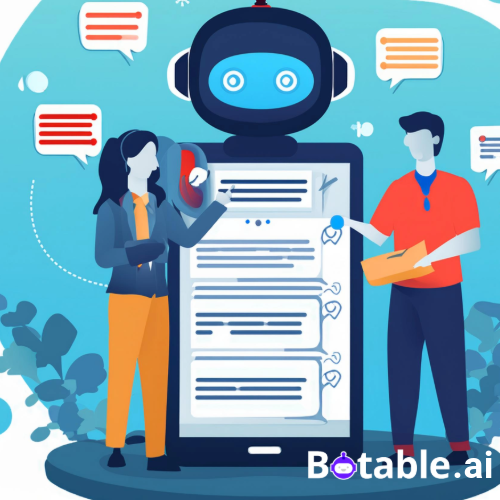
Future Directions and Competitive Advantages
As we look towards the horizon of AI-driven employee service, it's clear that chatbots equipped with sophisticated feedback loops will play a pivotal role in shaping the future of business-employee interactions. This section explores the emerging trends, potential advantages, and real-world examples of companies leveraging these technologies for strategic gain.
The future landscape: Predictions for AI chatbots and feedback loops
The evolution of AI chatbots and their feedback mechanisms will dramatically transform the employee service landscape. As these technologies advance, we can expect to see:
Emerging technologies enhancing feedback collection and analysis
- Advanced Natural Language Processing (NLP): Improved ability to understand context, sentiment, and nuance in employee feedback.
- Real-time sentiment analysis: Real-time monitoring and analytics can help identify issues as they occur, allowing for quick interventions to correct misunderstandings. This immediate response capability will significantly enhance user experience.
- Predictive analytics: AI systems that can anticipate employee needs based on historical data and current trends.
- Augmented Reality (AR) integration: Chatbots that can guide users through complex processes using visual overlays.
Hybrid models combining AI efficiency with human empathy
The future of chatbots lies in striking the perfect balance between AI efficiency and human touch. We can anticipate:
- Seamless handoffs between AI and human agents for complex queries.
- AI assistants can detect emotional cues and adjust their communication style accordingly.
- Collaborative AI-human teams where chatbots handle routine tasks, freeing human agents to focus on high-value, empathy-driven interactions.
Leveraging sophisticated feedback loops for strategic advantage
Feedback loops provide a competitive advantage by meeting evolving employee expectations and adapting to changing market trends[2]. Companies that effectively implement these systems can expect to:
- Gain deeper insights into employee preferences and pain points.
- Rapidly iterate and improve their products or services based on real-time feedback.
- Reduce operational costs while simultaneously improving employee satisfaction.
- Stay ahead of competitors by quickly adapting to market changes.
Building trust through improved employee interactions
As chatbots become more sophisticated, they have the potential to build stronger, trust-based relationships with employees. This can be achieved through:
- Personalized interactions based on employee history and preferences.
- Consistent and accurate responses that instill confidence in the AI system.
- Transparent communication about the chatbot's capabilities and limitations.
- Proactive problem-solving that anticipates and addresses employee needs before they arise.
Tagged:
Thinking about Botable for your team? See it live
.png)
In just 30 minutes, see how Botable can transform your workplace with a personalized demo.
Identify your unique challenges
Flexible pricing options
Easy integrations
Step-by-step implementation plan
Customize Botable for your workflow
Meet with the team



.png)