Full-Text Search AI: The Future of Quality Management
Data is the lifeblood of business operations and quickly and accurately sifting through vast amounts of information can make or break a company's quality management efforts. Enter Full-Text Search AI – a game-changing technology transforming how organizations approach quality control and assurance. By harnessing the power of artificial intelligence, businesses can unlock insights hidden within mountains of unstructured data, transforming their quality management systems from reactive to proactive powerhouses. This article delves into the transformative impact of Full Text Search AI on quality management, exploring how it's reshaping industries and setting new standards for efficiency and accuracy.
Introduction to Full-Text Search AI in Quality Management
Defining Full-Text Search and Its Relevance to Quality Management
Full Text Search is a sophisticated technique within search architecture that allows users to search for specific words, phrases, or patterns within large volumes of text data. Unlike traditional keyword searches, Full Text Search examines the entire content of documents, databases, or web pages, providing more comprehensive and relevant results. When powered by AI, this capability becomes even more potent, offering contextual understanding and semantic analysis that goes beyond simple word matching.
In the realm of quality management, Full Text Search AI is a game-changer. It enables quality professionals to rapidly sift through vast repositories of documentation, including product specifications, customer feedback, adverse event reports, and regulatory guidelines. This technology is particularly crucial in industries where compliance and precision are paramount, such as healthcare, manufacturing, and finance.
The relevance of Full Text Search AI to quality management cannot be overstated. It allows for:
- Rapid identification of quality issues across multiple data sources
- Efficient tracking of compliance with industry standards and regulations
- Quick retrieval of relevant historical data for trend analysis and continuous improvement
- Enhanced ability to correlate seemingly unrelated quality incidents
By leveraging Full-Text Search AI, quality management systems (QMS) can become more proactive, identifying potential issues before they escalate into major problems.
The Evolution of Quality Management Systems and the Integration of AI
Quality management systems have come a long way from their origins in statistical process control and Total Quality Management (TQM) philosophies. The digital transformation has ushered in a new era of data-driven quality management, where AI plays an increasingly central role.
The evolution of QMS can be broadly categorized into several phases:
- Manual documentation and inspection-based systems
- Computer-aided quality control with basic data analysis
- Integrated quality management software solutions
- Cloud-based QMS with real-time data processing
- AI-powered QMS with predictive analytics and Full Text Search capabilities
Integrating AI into quality management systems represents a quantum leap in capabilities. AI-driven Full Text Search is not just about finding information faster; it's about deriving actionable insights from complex, unstructured data sets. This technology enables quality managers to make more informed decisions, predict potential quality issues, and streamline processes across the entire quality management lifecycle.
Moreover, integrating AI into QMS has paved the way for more sophisticated analytics. Machine learning algorithms can now detect patterns and anomalies that human analysts might miss, leading to earlier detection of quality issues and more effective preventive measures. Natural Language Processing (NLP) capabilities enable systems to understand and categorize textual data from various sources, including customer complaints, audit reports, and supplier evaluations.
As quality management systems continue to evolve, the role of Full Text Search AI is becoming increasingly central. It's not just an add-on feature but a fundamental component that enhances every aspect of quality management, from document control to risk assessment and corrective action planning. The synergy between AI and quality management sets new benchmarks for operational excellence and customer satisfaction across industries.
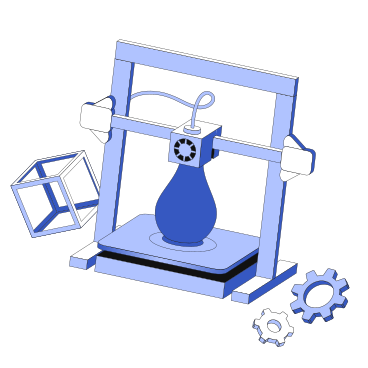
Traditional vs AI-powered full-text Searches in Quality Control
A Comparative Analysis Highlighting Accuracy, Speed, and Contextual Understanding
In quality control, the shift from traditional Full-Text Search methods to AI-powered solutions has significantly improved how organizations manage and utilize their data. This comparative analysis delves into the key differences between these two approaches, focusing on accuracy, speed, and contextual understanding.
Accuracy:
Traditional Full Text Search:
- It relies heavily on exact keyword matches
- Often struggles with synonyms and industry-specific terminology
- Can miss relevant results due to variations in phrasing or spelling
- Accuracy decreases with larger datasets
AI-Powered Full Text Search:
- Utilizes semantic understanding to interpret query intent
- Recognizes synonyms, acronyms, and domain-specific language
- Handles variations in phrasing and spelling with ease
- Maintains high accuracy even with vast amounts of data
- Learns and improves over time, increasing precision with use
Speed:
Traditional Full Text Search:
- Generally fast for simple queries on small to medium-sized datasets
- Performance degrades with complex queries or large volumes of data
- Requires manual optimization for maintaining speed as data grows
AI-Powered Full Text Search:
- Delivers rapid results even for complex queries on massive datasets
- Utilizes advanced indexing and data structures for efficient retrieval
- Scales seamlessly to accommodate growing data volumes
- Employs predictive caching to anticipate and pre-load likely searches
Contextual Understanding:
Traditional Full Text Search:
- Limited to literal interpretation of search terms
- Struggles to understand the context or intent behind queries
- Often returns irrelevant results due to lack of contextual awareness
AI-Powered Full Text Search:
- Employs Natural Language Processing to understand query context
- Interprets user intent, even with ambiguous or poorly formulated queries
- Provides contextually relevant results, improving the quality of information retrieved
- Adapts to user behavior and preferences over time
In quality control applications, these differences translate to tangible benefits:
- Enhanced Precision: AI-powered Full Text Search significantly reduces false positives and negatives in quality-related searches, ensuring that critical issues are not overlooked.
- Time Efficiency: The speed and accuracy of AI-driven searches allow quality control teams to quickly access relevant information, reducing response times to quality issues.
- Improved Decision Making: By providing contextually relevant results, AI-powered searches enable quality control professionals to make more informed decisions based on comprehensive data.
- Proactive Quality Management: The ability of AI to understand trends and patterns in search behavior can help organizations identify potential quality issues before they escalate.
- Scalability: As organizations accumulate more quality-related data, AI-powered Full Text Search maintains its performance, ensuring consistent efficiency regardless of data volume.
While traditional Full Text Search methods have served quality control processes for years, the advent of AI-powered solutions represents a significant leap forward. The enhanced accuracy, speed, and contextual understanding offered by AI not only improve the efficiency of quality control operations but also enable a more proactive and comprehensive approach to quality management.
As AI technology continues to evolve, we can expect even greater advancements in Full Text Search capabilities, further widening the gap between traditional and AI-powered methods. Organizations that embrace these AI-driven solutions in their quality control processes are likely to gain a competitive edge through improved efficiency, reduced errors, and more strategic quality management practices.

The Power of AI in Enhancing Full-Text Search Capabilities
Detailed Overview of How AI Redefines Text Analytics and NLP
Artificial Intelligence (AI) has revolutionized the landscape of text analytics and Natural Language Processing (NLP), bringing unprecedented power to Full Text Search capabilities. Integrating AI into these domains has transformed how we interact with and extract value from textual data, particularly in the context of quality management.
AI-driven text analytics goes beyond simple keyword matching, employing sophisticated algorithms to understand the context, sentiment, and intent behind the text. This advanced comprehension allows for more nuanced and accurate analysis of quality-related documents, customer feedback, and operational reports.
In the realm of NLP, AI has made significant strides in:
- Entity Recognition: Identifying and categorizing key elements within text, such as product names, defect types, or regulatory standards.
- Sentiment Analysis: Gauging the tone and emotional content of text data, crucial for interpreting customer feedback and internal communications.
- Topic Modeling: Automatically discovering abstract topics within large collections of documents, helping to organize and categorize vast amounts of quality-related information.
- Language Translation: Breaking down language barriers in global quality management operations by providing accurate, context-aware translations.
These advancements in AI-powered text analytics and NLP have dramatically enhanced the capabilities of Full Text Search, making it an indispensable tool in modern quality management systems.
{{cta}}
Benefits of Full-Text Search AI Over Traditional Search Methods in Quality Data Analysis
Integrating AI into Full Text Search offers numerous advantages over traditional search methods, particularly regarding quality data analysis. These benefits are transforming how organizations approach quality management, enabling more efficient and effective processes.
- Enhanced Contextual Understanding
- Improved Accuracy and Relevance
AI algorithms can learn from user interactions and feedback, continuously refining search results. This leads to increasingly accurate and relevant outcomes over time, reducing the noise in search results and helping quality professionals quickly find the exact information they need.
Handling of Unstructured Data:
Traditional search methods often struggle with unstructured data, which is prevalent in quality management (e.g., free-text comments, incident reports, or customer reviews). AI-powered Full Text Search analyzes and extracts insights from unstructured data, providing a more comprehensive view of quality-related information.
Predictive Analytics:
By analyzing patterns and trends in historical quality data, AI can offer predictive insights, helping organizations anticipate potential quality issues before they occur. This proactive approach is a significant advancement over-reactive traditional methods.
Natural Language Querying:
AI enables users to interact with search systems using natural language queries, making it easier for quality professionals to find information without needing to construct complex search strings or learn specialized query languages.
Multi-lingual Support:
AI-powered Full-Text Search can effectively handle multiple languages, which is crucial for global organizations managing quality across different regions and markets.
Automated Categorization and Tagging:
AI can automatically categorize and tag documents based on their content, making it easier to organize and retrieve quality-related information without manual intervention.
Continuous Learning and Improvement:
Unlike static traditional search methods, AI-powered systems continuously learn and improve from user interactions, adapting to changing terminology and emerging quality concerns over time.
In conclusion, the power of AI in enhancing Full-Text Search capabilities represents a significant leap forward in quality data analysis. By leveraging advanced text analytics, NLP, and machine learning, AI-powered Full Text Search offers insight and efficiency that traditional methods simply cannot match. As organizations grapple with increasing volumes of quality-related data, the role of AI in Full Text Search will only become more critical, driving improvements in quality management across industries.
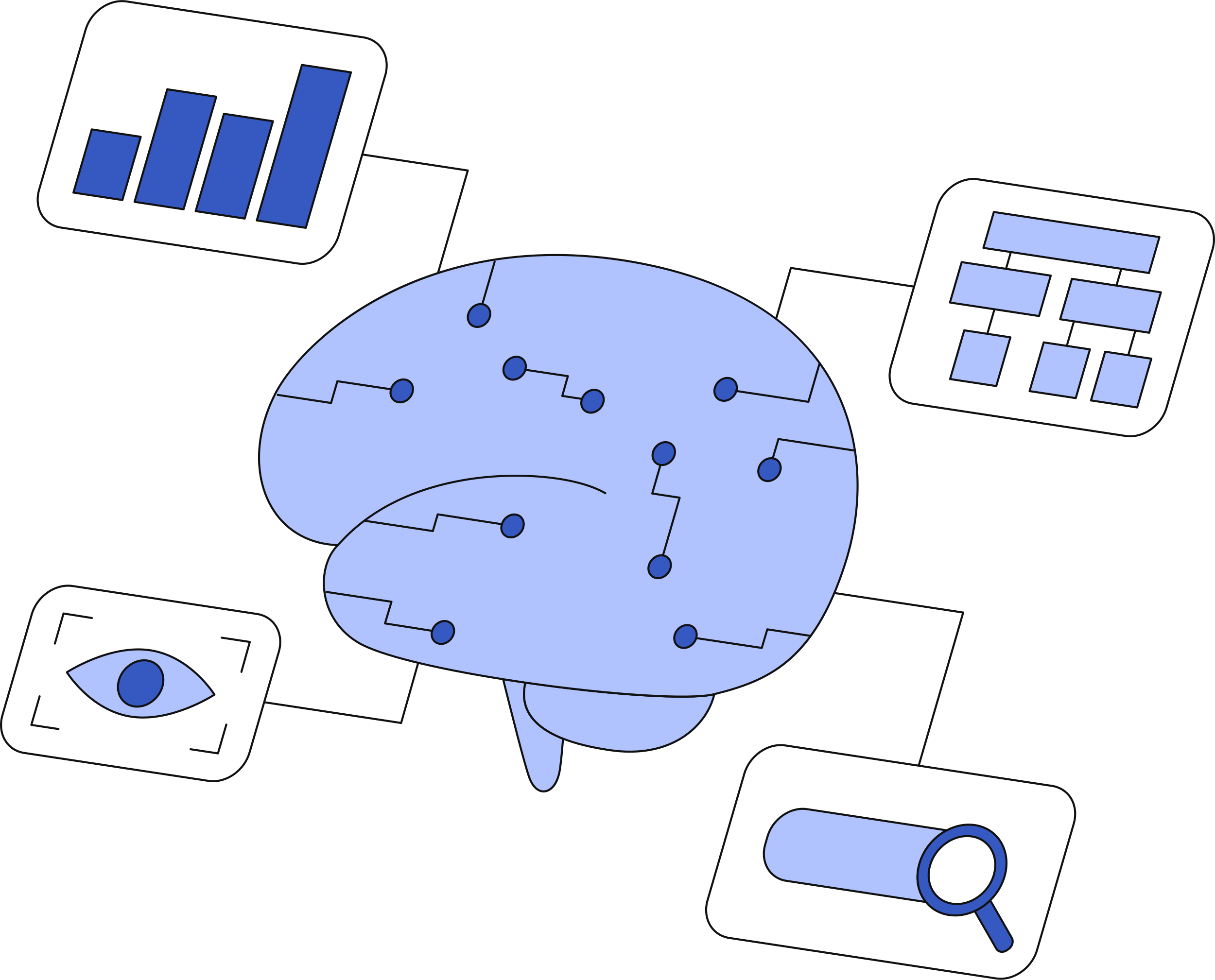
Core Components of Full-Text Search AI for Optimum Quality Management
Semantic Analysis: The Backbone of Relevance in AI-powered Searches
Semantic analysis forms the cornerstone of AI-powered Full Text Search systems, enabling them to understand the context and meaning behind user queries and document content. This sophisticated component goes beyond simple keyword matching, delving into the intricacies of language to deliver highly relevant results in quality management scenarios.
At its core, semantic analysis in Full Text Search AI involves:
- Natural Language Understanding (NLU): This allows the system to interpret the intent behind user queries, even when they're phrased in colloquial or industry-specific language.
- Entity Recognition: Identifying and categorizing key elements within text, such as product names, quality standards, or regulatory bodies.
- Concept Extraction: Recognizing abstract ideas and themes within documents, which is crucial for connecting related quality management concepts across different texts.
Moreover, semantic analysis enables the AI to understand synonyms, acronyms, and industry-specific terminology, ensuring that searches return comprehensive results even when exact terms aren't used. This is particularly valuable in quality management, where precision and completeness of information are paramount.
Innovative Indexing and Data Structures for Efficient Information Retrieval
The efficiency of Full Text Search AI in quality management heavily relies on innovative indexing techniques and data structures. These components work behind the scenes to organize vast amounts of quality-related data for rapid retrieval and analysis.
Key aspects of this component include:
- Inverted Indexing: This technique maps words to their locations in documents, allowing for quick lookups and efficient searching across large datasets.
- Vector Space Models: These models represent documents and queries as vectors in a high-dimensional space, enabling similarity comparisons and ranking of search results.
- Probabilistic Models: These use statistical methods to estimate the likelihood of a document being relevant to a given query, improving search accuracy.
- Hierarchical Data Structures: Organizing data in tree-like structures allows for faster navigation and retrieval of information, particularly useful for handling complex quality management documentation.
- Distributed Indexing: For organizations with massive amounts of quality data, distributed indexing across multiple servers ensures scalability and maintains search speed.
The combination of semantic analysis and innovative indexing creates a powerful Full Text Search AI system capable of:
- Rapidly retrieving relevant quality documents from vast repositories
- Identifying connections between seemingly unrelated quality issues
- Providing contextually accurate results to complex quality management queries
- Scaling to accommodate growing volumes of quality-related data without compromising speed
By leveraging these core components, Full Text Search AI significantly enhances the efficiency and effectiveness of quality management processes. It enables organizations to access critical information quickly, proactively identify potential quality issues, and comply with ever-evolving industry standards.
As AI technology continues to advance, we can expect even more sophisticated semantic analysis and indexing techniques to emerge, further refining the capabilities of Full Text Search in quality management. This ongoing evolution will empower organizations to achieve new operational excellence and quality assurance levels.
The Strategic Implementation of Full-Text Search AI in Quality Assurance Processes
Best Practices for Incorporating AI into Existing Quality Management Frameworks
Integrating Full Text Search AI into existing Quality Management Systems (QMS) requires a strategic approach to ensure seamless adoption and maximum benefit. Here are some best practices for incorporating this powerful technology:
- Assess Current QMS Capabilities: Begin by evaluating your existing QMS to identify areas where Full Text Search AI can add the most value. Look for processes that involve extensive document searches or data analysis.
- Define Clear Objectives: Establish specific goals for implementing Full Text Search AI, such as reducing search time, improving accuracy in document retrieval, or enhancing compliance monitoring.
- Start with Pilot Projects: Implement AI-powered Full-Text Search in a controlled environment or specific department before rolling it out company-wide. This allows for fine-tuning and demonstrating value.
- Ensure Data Quality and Standardization: AI systems rely on high-quality, standardized data. Review and clean your existing data, and establish protocols for maintaining data integrity moving forward.
- Integrate with Existing Systems: Ensure that the AI-powered Full-Text Search solution can seamlessly integrate with your current QMS and other relevant software systems.
- Provide Comprehensive Training: Using AI, quality managers can access necessary documents quickly, even without recalling exact document codes, enhancing productivity and reducing errors. To achieve this, invest in thorough training programs for all users to ensure they can leverage the full potential of the AI system.
- Establish Governance and Oversight: Develop clear guidelines for the use of AI in your QMS, including data privacy considerations, ethical use policies, and regular audits of AI performance.
- Continuous Monitoring and Improvement: Regularly assess the performance of your AI-powered Full-Text Search system and gather user feedback to drive continuous improvements.
- Leverage AI Insights for Process Improvement: Use the insights generated by AI to identify trends, potential quality issues, and areas for process improvement within your QMS.
Adapting to the Technological Shift: Skills and Infrastructure Requirements
Implementing Full Text Search AI in quality assurance processes necessitates adaptations in both skills and infrastructure. Here's what organizations need to consider:
Skills Requirements:
- AI Literacy: Develop a workforce that understands the basics of AI and its applications in quality management. This includes both technical and non-technical staff.
- Data Analysis Skills: Enhance data analysis capabilities within your quality assurance team to interpret and act on AI-generated insights.
- Technical Expertise: Build or acquire expertise in AI technologies, natural language processing, and machine learning to support and maintain your AI systems.
- Change Management Skills: Cultivate change management skills to facilitate the smooth adoption of AI technologies across the organization.
- Ethical AI Understanding: Ensure key personnel are trained in the ethical considerations of AI use, including data privacy and bias mitigation.
Infrastructure Requirements:
- Robust Data Infrastructure: Implement a scalable and secure infrastructure capable of handling large volumes of structured and unstructured data.
- Cloud Computing Resources: Consider cloud-based solutions to provide the necessary computing power and storage for AI-powered Full Text Search systems.
- Integration Capabilities: Ensure your infrastructure supports seamless integration between AI systems, existing QMS, and other enterprise software.
- Security Measures: Implement robust cybersecurity measures to protect sensitive quality-related data processed by AI systems.
- User-Friendly Interfaces: Develop intuitive user interfaces that allow quality professionals to interact with AI-powered search tools easily.
Organizations can successfully implement Full Text Search AI into their quality assurance processes by following these best practices and addressing the skills and infrastructure requirements. This strategic approach enhances the efficiency of quality management and positions the organization to adapt to future technological advancements in AI and quality assurance.

Challenges and Considerations in Employing Full-Text Search AI for Quality Control
While the benefits of implementing Full-Text Search AI in quality control are substantial, organizations must navigate several challenges and considerations to ensure successful adoption and compliance. This section explores managing the transition from traditional methods to AI-enabled processes.
Managing the Transition: From Traditional Methods to AI-enabled Processes
Transitioning from traditional quality control methods to AI-enabled processes requires careful planning and execution. Here are vital considerations for managing this transition effectively:
- Phased Implementation: Adopt a phased approach, gradually introducing AI-powered Full Text Search capabilities alongside existing systems to minimize disruption and allow for adjustment.
- Data Integration: AI in big data quality management can automate data cleansing, normalization, and deduplication, ensuring real-time monitoring and automated anomaly detection to maintain high-quality data. Organizations should leverage these capabilities to integrate disparate data sources and ensure data quality before full AI implementation.
- Training and Skill Development: Invest in comprehensive training programs to upskill employees in using AI-powered Full Text Search tools and interpreting AI-generated insights.
- Change Management: Implement a robust change management strategy to address resistance and ensure buy-in from all stakeholders in the quality control process.
- Performance Benchmarking: Establish clear metrics to measure the performance of AI-enabled processes against traditional methods, ensuring tangible improvements in efficiency and accuracy.
- Hybrid Approaches: Consider hybrid approaches that combine the strengths of traditional methods with AI capabilities, especially in critical quality control processes where human oversight remains crucial.
- Continuous Evaluation: Regularly assess the effectiveness of AI-powered Full Text Search in quality control, gathering user feedback and adjusting the system as needed.
- Ethical Considerations: Develop guidelines for the ethical use of AI in quality control, addressing concerns about job displacement and ensuring that AI augments rather than replaces human expertise.
- Scalability Planning: Design the transition with scalability in mind, ensuring that the AI-powered Full Text Search system can grow with the organization's needs and adapt to future technological advancements.
- Vendor Selection and Partnership: Carefully select AI technology vendors, prioritizing those with experience in quality control applications and a commitment to ongoing support and development.
Organizations can navigate the complexities of implementing Full-Text Search AI in quality control processes by addressing these challenges and considerations. The transition requires a balanced approach that leverages the power of AI while maintaining robust data privacy practices and ensuring a smooth transition for all stakeholders involved. As AI technologies evolve, staying informed and adaptable will be key to maximizing the benefits of Full Text Search AI in quality control while mitigating potential risks.
Maximizing the Value of Full-Text Search AI in Ensuring Optimal Quality Standards
As we've explored throughout this article, the integration of AI and Full Text Search capabilities into Quality Management Systems (QMS) represents a significant leap forward in ensuring optimal quality standards across industries. Organizations must adopt a strategic and holistic approach to maximize the value of these technologies.
First and foremost, it's crucial to recognize that Full Text Search AI is not just a tool for faster document retrieval; it's a catalyst for transforming entire quality management processes. By leveraging AI's ability to understand context, identify patterns, and predict potential issues, companies can shift from reactive to proactive quality management strategies.
To truly maximize the value of Full Text Search AI in QMS, consider the following key points:
- Data Integration and Quality: Ensure that your AI-powered Full Text Search system has access to comprehensive, high-quality data from all relevant sources within your organization. The effectiveness of AI is directly proportional to the quality and breadth of data it can analyze.
- Continuous Learning and Improvement: Implement feedback loops that allow your AI system to learn from user interactions and quality outcomes. This continuous improvement cycle will enhance the accuracy and relevance of search results over time.
- Cross-functional Collaboration: Encourage collaboration between quality management teams, IT departments, and data scientists. This interdisciplinary approach will ensure that the Full Text Search AI system is aligned with both technical capabilities and quality management objectives.
- User-Centric Design: Design the AI-powered search interface with the end-users in mind. An intuitive, user-friendly system will encourage adoption and maximize the value derived from the technology.
- Ethical and Responsible AI Use: Develop clear guidelines for the ethical use of AI in quality management. This includes addressing issues of bias, transparency, and data privacy to build trust in the system.
- Scalability and Flexibility: Choose AI and Full Text Search solutions that can scale with your organization's growth and adapt to changing quality management needs and regulatory requirements.
- Performance Metrics and ROI Tracking: Establish clear metrics to measure the impact of Full Text Search AI on your quality management processes. This will help justify investments and identify areas for further improvement.
- Training and Change Management: Invest in comprehensive training programs to ensure that all stakeholders can effectively utilize the AI-powered search capabilities. A well-executed change management strategy is crucial for successful adoption.
- Integration with Existing QMS: Seamlessly integrate the Full Text Search AI capabilities with your existing QMS to create a cohesive and efficient quality management ecosystem.
- Future-Proofing: Stay informed about emerging AI technologies and their potential applications in quality management. Regularly assess and update your Full Text Search AI system to leverage new capabilities as they become available.
By focusing on these aspects, organizations can unlock the full potential of Full Text Search AI in their quality management processes. The result is a more agile, efficient, and effective QMS that can adapt to the complexities of modern business environments.
As we look to the future, it's clear that AI and Full Text Search will play an increasingly central role in maintaining and improving quality standards across industries. Those who embrace these technologies and integrate them thoughtfully into their QMS will be well-positioned to lead in their respective fields, ensuring not just compliance with quality standards, but the continuous enhancement of product and service quality.
In conclusion, the value of Full Text Search AI in ensuring optimal quality standards is immense and multifaceted. By approaching its implementation strategically and holistically, organizations can transform their quality management processes, driving efficiency, accuracy, and innovation. As AI continues to evolve, so too will its capabilities in quality management, promising an exciting future where quality assurance is more proactive, precise, and powerful than ever before.
What is Fuzzy Search?
Fuzzy Search in the context of AI chatbots, or more broadly in information retrieval systems, refers to a method where the search algorithm allows for mistakes or variations in the input query. The primary goal of fuzzy search is to find matches even when the user's input isn't exactly matched for what's in the database. This accounts for typos, misspellings, or slight variations in how terms might be entered.
What is a traditional full-text search example?
Consider a vast database containing millions of documents. An example of a full-text search would be querying this database for the term "temperature control".
The search engine scans every document in its repository to find instances of "temperature control". This includes not just titles or keywords but the entire text within each document.
The engine retrieves and displays all relevant documents where “temperature control” appears in an instant, ensuring a comprehensive search outcome. Advanced algorithms ensure high speed and accuracy.
This capability is especially valuable in sectors like legal, academic, and scientific research, where precision and thoroughness are paramount. Full-text search AI ensures no critical information is overlooked.
Harnessing full-text search AI, we unlock unparalleled efficiency and depth, revolutionizing how we access and utilize vast information troves.
What is the difference between full-text search and semantic search?
Full-text search queries a large volume of text to locate exact matches of user-provided keywords or phrases. It relies heavily on indexing and pattern matching and focuses primarily on textual content. AI can enhance this to find relevant but not exactly matching items.
In contrast, semantic search aims to understand the context and meaning behind the words used. This approach leverages advanced natural language processing (NLP) and machine learning algorithms to comprehend the intent behind a query.
While full-text search delivers precise results by matching specific terms, it can miss relevant documents that use different wording. Conversely, semantic search excels in grasping concepts and retrieving information based on inferred meaning.
For instance, searching for “temperature control” in a full-text system may not yield “thermometer” unless both terms are indexed. However, a semantic search would recognize the synonymy and fetch the relevant documents regardless of the precise term used.
The sophistication of semantic search results in a richer, more nuanced user experience, allowing for deeper and more accurate information retrieval. It transcends mere keyword matching and ventures into understanding context, user intent, and conceptual relationships.
As organizations seek to enhance their data retrieval capabilities, integrating both full-text and semantic search offers a powerful synergy. This combination ensures precision while harnessing the depth of contextual understanding that modern AI technologies provide.
Answers your employees need, right when they need them
Meet Botable — the AI chatbot that handles everything from simple FAQs to complex, multi-step questions, so your team can focus on what matters. Built for HR, QA, and beyond.
Continue reading
Ready to see what Botable can do for you?
Book your demo now to see how Botable can transform your workplace.
Identify your unique challenges
Flexible pricing options
Easy integrations
Step-by-step implementation plan
Customize Botable for your workflow
Book a demo
.webp)