AI-Driven Root Cause Analysis: Transform Quality Incidents
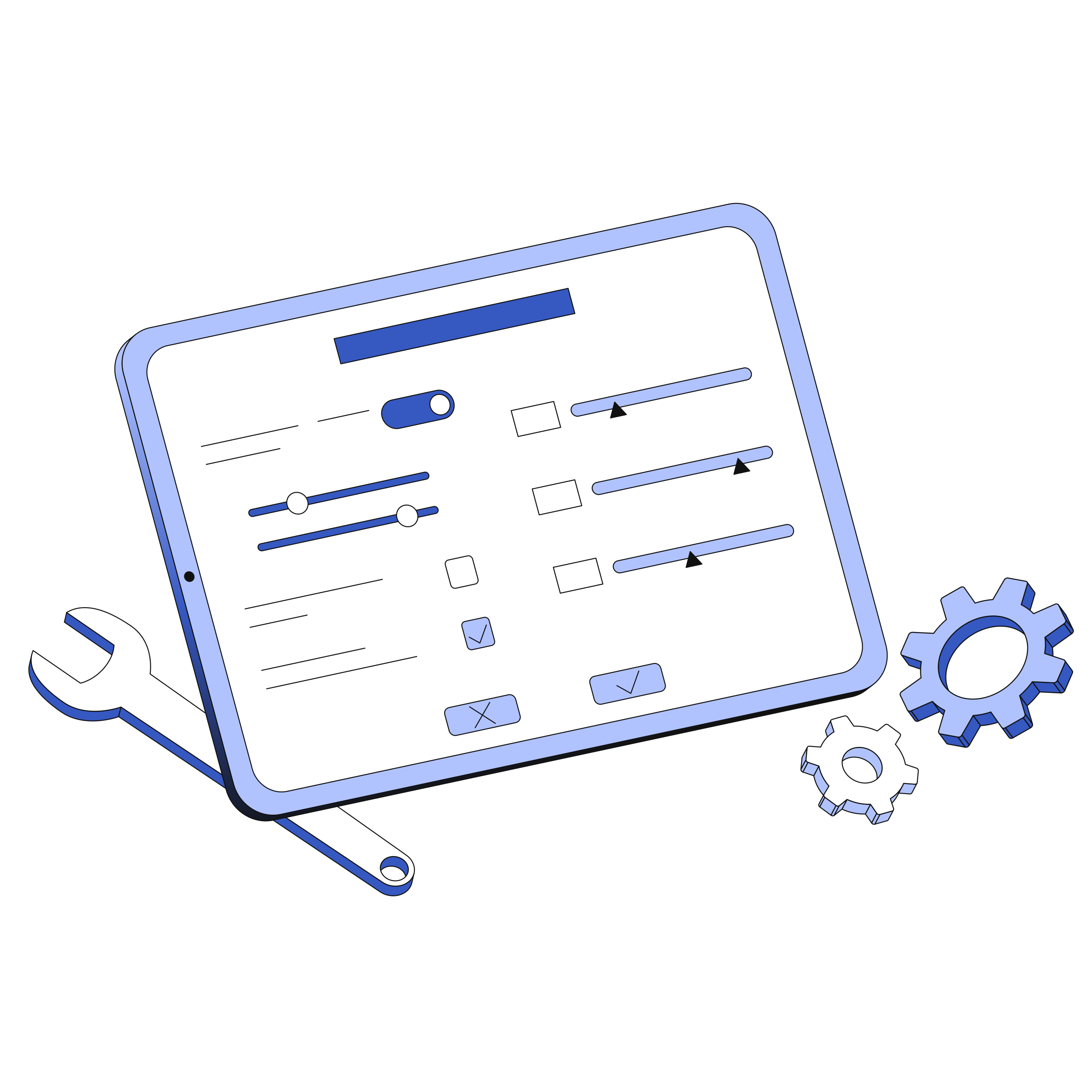
In the fast-paced manufacturing world, where precision and efficiency reign supreme, a single quality incident can send ripples through an entire production line. But what if we could harness the power of artificial intelligence to identify these issues and uncover their root causes with unprecedented accuracy and speed? Welcome to the era of AI-driven root cause analysis – a game-changing approach that's revolutionizing how we tackle quality incidents in manufacturing through generative AI.
Introduction: Unveiling the Potential of AI in Root Cause Analysis for Quality Incidents
In recent years, the manufacturing sector has witnessed a seismic shift in its approach to quality control and incident management, driven by the increasing adoption of automation. Integrating artificial intelligence into root cause analysis (RCA) processes is at the forefront of this transformation. This innovative fusion of technology and analytical methodology promises to reshape the quality management landscape, offering manufacturers unprecedented insights and efficiency in addressing quality incidents.
Introduction: The Importance of RCA in Manufacturing
Root cause analysis, complemented by diagnostics, is a cornerstone of quality management in the manufacturing industry. Its significance cannot be overstated, as it is the primary tool for identifying the underlying causes of quality issues, defects, and production anomalies. By pinpointing the root cause of a problem, manufacturers can implement targeted solutions, preventing recurrence and enhancing overall product quality.
In the competitive landscape of modern manufacturing, where margins are tight and customer expectations are high, effective RCA is not just beneficial – it's essential. It enables companies to minimize downtime, reduce waste, and maintain their reputation for quality and reliability. Moreover, a robust RCA process contributes to continuous improvement initiatives, fostering a culture of learning and adaptation within the organization.
The impact of RCA extends beyond immediate problem-solving. It plays a crucial role in:
- Improving product design and manufacturing processes
- Enhancing safety protocols and reducing workplace incidents
- Optimizing resource allocation and reducing operational costs
- Strengthening compliance with industry standards and regulations
As manufacturing processes become increasingly complex and interconnected, the need for sophisticated RCA methods has never been more pressing. AI-driven approaches are making a significant impact in this area, offering new possibilities for deeper, faster, and more accurate analysis.
Traditional Methods of RCA and Their Limitations
Traditionally, root cause analysis in manufacturing has relied on a combination of human expertise and structured problem-solving methodologies. Common approaches include the "5 Whys" technique, fishbone diagrams (Ishikawa diagrams), and fault tree analysis. While these methods have served the industry well for decades, they come with inherent limitations that can hinder their effectiveness in today's fast-paced and data-rich manufacturing environments.
One of the primary challenges of traditional RCA methods is their reliance on human judgment and experience, which can contribute to human error. While invaluable, human expertise can be subject to biases, inconsistencies, and limitations in processing vast data. This can lead to oversights in complex scenarios or situations where the root cause is not immediately apparent.
Moreover, traditional approaches often struggle with:
- Time constraints: Manual analysis can be time-consuming, delaying critical decisions and corrective actions.
- Data volume and complexity: Modern manufacturing generates enormous amounts of data from various sources, which can overwhelm traditional analysis methods.
- Interconnected systems: As manufacturing processes become more integrated, identifying the root cause in a complex web of interrelated factors becomes increasingly challenging.
- Scalability: Traditional methods may not scale effectively across multiple production lines or facilities.
These limitations highlight the need for more advanced, data-driven approaches to RCA. As manufacturing continues to evolve, so must the tools and methodologies to ensure quality and efficiency. This is where AI-driven root cause analysis enters the picture, offering a powerful solution to overcome these challenges and transform how quality incidents are analyzed and resolved.
By 2025, AI is predicted to be a top-five investment priority for more than 30% of CIOs. This statistic underscores the growing recognition of AI's potential to revolutionize various aspects of business operations, including quality management and root cause analysis in manufacturing. As we delve deeper into the capabilities of AI-driven RCA, it becomes clear that this technology is not just a prospect but a present reality reshaping the manufacturing landscape.
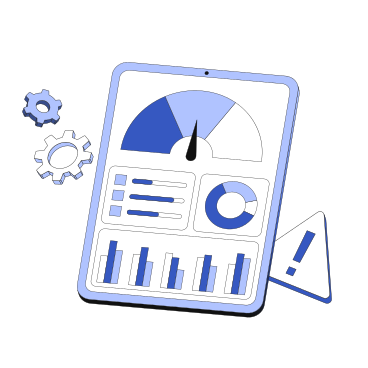
Depth Look at AI Technologies Powering RCA
AI-driven root cause analysis is revolutionizing the manufacturing industry, offering unprecedented capabilities in identifying and resolving quality incidents. At the heart of this transformation are two key concepts: automated root cause analysis and automatic root cause analysis. While these terms are often used interchangeably, they represent the core of how AI is reshaping traditional RCA processes.
Automated root cause analysis leverages AI algorithms to streamline the process of identifying the underlying causes of quality issues. This approach significantly reduces the manual effort required in traditional RCA methods, allowing faster and more efficient problem-solving. On the other hand, automatic root cause analysis takes this further by employing machine learning models that can independently analyze data and draw conclusions with minimal human intervention.
The power of these AI-driven approaches is evident in their performance metrics. AI-powered RCA can reduce the time spent on root cause analysis by up to 70%. This dramatic reduction in analysis time allows manufacturers to respond to quality incidents more swiftly, minimizing downtime and potential financial losses.
Moreover, the accuracy of AI-powered RCA is remarkable. AI-powered RCA in complex manufacturing systems achieved an accuracy of 95%, compared to 78% for traditional statistical methods. This increased accuracy translates to more reliable solutions and fewer recurring issues, ultimately leading to improved product quality and customer satisfaction.
Another key advantage is AI's efficiency in processing vast amounts of data. AI-powered RCA can process vast amounts of data 10 times faster than traditional methods. This capability allows manufacturers to analyze complex, interconnected systems and identify root causes that conventional approaches might overlook.
Some of the key AI technologies powering automated and automatic root cause analysis include:
- Machine Learning Algorithms: These algorithms learn from historical data to identify patterns and predict potential root causes of quality issues.
- Natural Language Processing (NLP): NLP enables the analysis of unstructured data from maintenance logs, operator reports, and customer feedback to extract valuable insights.
- Computer Vision: This technology can analyze visual data from production lines, identifying defects and anomalies that may contribute to quality incidents.
- Deep Learning Networks: These advanced neural networks can handle complex, non-linear relationships in manufacturing processes, uncovering hidden root causes.
- Predictive Analytics: By analyzing historical and real-time data, predictive analytics can forecast potential quality issues before they occur, enabling proactive measures.
The scalability of AI-powered RCA is another significant advantage. The scalability of AI-powered RCA is rated 9 out of 10, compared to 3 out of 10 for traditional methods. This means that as manufacturing operations grow and become more complex, AI-driven RCA can easily adapt and maintain its effectiveness across multiple production lines or facilities.
In conclusion, the depth and breadth of AI technologies powering automated and automatic root cause analysis are transforming the manufacturing quality management landscape. By harnessing these advanced capabilities, manufacturers can achieve unprecedented efficiency, accuracy, and scalability in their RCA processes, ultimately improving product quality and operational excellence.
{{cta}}
Conversational Root Cause Analysis Using Chatbots
In the evolving landscape of AI-driven root cause analysis, a new player has emerged, revolutionizing how manufacturers approach quality incidents: conversational AI chatbots. These intelligent virtual assistants, powered by generative AI, are transforming how teams interact with data and conduct root cause analysis, making the process more intuitive, accessible, and efficient.
Chatbots designed for root cause analysis bring a human-like interface to complex analytical processes, enhancing diagnostics and minimizing human error through automation. They can engage in natural language conversations with users, guiding them through the analysis process step-by-step. This conversational approach democratizes the RCA process, allowing team members at various levels of technical expertise to contribute effectively to problem-solving efforts.
One company at the forefront of this technology is Botable, an AI-powered QMS chatbot for manufacturing. Botable's technology exemplifies the potential of conversational AI in various business processes, including root cause analysis in manufacturing.
Botable's chatbots learn a company's specific information, such as employee documentation and policies. Similarly, in a manufacturing context, RCA chatbots can be trained on a company's quality control procedures, historical incident data, and best practices for problem-solving. This allows the chatbot to provide contextually relevant guidance during the root cause analysis.
The integration capabilities of chatbots like Botable are particularly relevant for RCA applications. Botable seamlessly integrates with thousands of enterprise tools and platforms, dramatically reducing setup time and double work for maximum efficiency and effectiveness. In the context of root cause analysis, this means that an RCA chatbot could potentially:
- Access real-time production data from manufacturing execution systems (MES)
- Pull historical quality incident reports from quality management systems (QMS)
- Retrieve maintenance logs from computerized maintenance management systems (CMMS)
- Analyze data from Internet of Things (IoT) sensors on the production line
By leveraging these integrations, an RCA chatbot can provide a comprehensive view of the factors contributing to a quality incident, all through a conversational interface.
The benefits of using chatbots for root cause analysis include:
- Faster problem-solving: Chatbots can quickly sift through vast amounts of data and guide users to relevant information, speeding up the RCA process.
- Consistency: Chatbots ensure that the RCA process follows a consistent methodology every time, reducing variability in analysis quality.
- Knowledge retention: As chatbots learn from each interaction, they build a knowledge base that can be applied to future incidents, improving the organization's problem-solving capabilities.
- 24/7 availability: Unlike human experts, chatbots are available 24/7 to assist with RCA, enabling immediate response to quality incidents.
Botable's approach demonstrates the potential for AI-powered chatbots in various business processes. As manufacturers look to implement conversational root cause analysis using chatbots, they can draw inspiration from solutions like Botable, adapting the technology to meet the specific needs of quality management and incident resolution in manufacturing environments.
By embracing conversational AI for root cause analysis, manufacturers can take a more collaborative, efficient, and effective approach to resolving quality incidents, ultimately improving product quality and operational excellence.

Step-by-Step Guide: Implementing Chatbot-Driven RCA in Your Operations
Implementing a chatbot-driven root cause analysis (RCA) system in your manufacturing operations can significantly enhance your quality management processes. While the concept may seem daunting, breaking it down into manageable steps can make the transition smoother and more effective. Here's a comprehensive guide to help you integrate this innovative technology into your operations:
Assess Your Current RCA Process
Begin by evaluating your existing root cause analysis procedures. Identify pain points, inefficiencies, and areas where a chatbot could add value. This assessment will help you set clear objectives for your chatbot implementation.
Define Your Requirements
Based on your assessment, outline the specific features and capabilities you need in your RCA chatbot. Consider data integration, analysis capabilities, and user interface preferences.
Choose the Right Platform
Select a chatbot platform that aligns with your requirements. Botable specializes in QMS chatbots, and their approach demonstrates the potential of AI-powered chatbots in business processes. Look for platforms that offer flexibility and integration capabilities tailored to manufacturing and quality management contexts.
Gather and Prepare Your Data
Collect all relevant data sources for your RCA process. This may include:
- Historical quality incident reports
- Production data from your MES
- Maintenance logs
- Standard operating procedures
- Best practices for problem-solving
Ensure this data is clean, structured, and ready for integration with your chatbot system.
Train Your Chatbot
Feed your prepared data into the chatbot system. As Botable's QMS chatbots learn from employee documentation and quality policies, your RCA chatbot should be trained on your specific quality management procedures and historical incident data.
Integrate with Existing Systems
Leverage the integration capabilities demonstrated by platforms like Botable to connect your RCA chatbot with your existing manufacturing systems. This might include your MES, QMS, CMMS, and IoT sensors on the production line.
Design the Conversation Flow
Create a logical, user-friendly conversation flow for your chatbot. This should guide users through the RCA process, asking relevant questions and providing insights based on the data analysis, including identifying potential causes related to human error.
Test and Refine
Before full deployment, conduct thorough testing of your chatbot. Simulate various quality incidents and ensure the chatbot provides accurate, helpful guidance, incorporating diagnostics to fine-tune its responses. Based on these test results, refine the system.
Train Your Team
Provide comprehensive training to your team on effectively interacting with the RCA chatbot. Emphasize that the chatbot is a tool to enhance their expertise, not replace it.
Deploy and Monitor
If possible, roll out your chatbot-driven RCA system, starting with a pilot group. Closely monitor its performance and gather feedback from users.
Continuous Improvement
Review the chatbot's performance and user feedback regularly. Use these insights to continuously improve the system, updating its knowledge base and refining its conversation flows.
Following these steps, you can implement an automation-driven, chatbot-powered RCA system in your manufacturing operations.
Remember, the goal is to create a system that enhances your team's problem-solving capabilities, speeds up the RCA process, and ultimately improves product quality and operational efficiency. With careful planning and implementation, a chatbot-driven RCA system can become an invaluable asset in your quality management toolkit.

Harnessing the Power of AI for Revolutionary Improvements in Quality Incident Management
Integrating AI-driven root cause analysis into quality incident management is not just an incremental improvement—it's a revolutionary leap forward. By harnessing the power of artificial intelligence, manufacturers can transform their approach to quality control, dramatically reducing downtime, improving efficiency, and enhancing overall product quality.
One of AI's most significant impacts on quality incident management is its ability to minimize costly downtime. The average cost of downtime for businesses can reach up to $260,000 per hour. With such high stakes, the speed and accuracy of AI-driven RCA become crucial. Manufacturers can significantly reduce these costly interruptions by quickly identifying and addressing the root causes of quality incidents.
The efficiency gains from AI-powered RCA are substantial. AI-driven systems have been shown to reduce the average time to resolve incidents by up to 50%. This dramatic reduction in resolution time means that production lines can return to total capacity faster, minimizing the impact of quality incidents on overall productivity.
Resource allocation is another area where AI demonstrates its transformative potential. AI-powered RCA can improve resource allocation efficiency from 60% to 85%. This improvement means that personnel, equipment, and materials are utilized more effectively in addressing quality issues, leading to cost savings and increased operational efficiency.
Perhaps one of the most exciting applications of AI in quality incident management is predictive maintenance. Using AI-powered RCA, predictive maintenance accuracy can be improved from 70% to 90%. This increased accuracy allows manufacturers to address potential issues before they escalate into full-blown quality incidents, further reducing downtime and maintenance costs.
The revolutionary improvements brought about by AI in quality incident management include:
Proactive Issue Detection:
AI algorithms can analyze patterns in production data to identify potential quality issues before they manifest, enabling preemptive action.
Continuous Learning:
AI systems continuously learn from each incident, improving their accuracy and effectiveness.
Holistic Analysis:
AI can consider a vast array of factors simultaneously, providing a more comprehensive understanding of quality incidents than traditional methods.
Real-time Insights:
AI-powered systems can provide instant analysis and recommendations, enabling rapid response to quality issues.
Enhanced Decision-making:
By providing data-driven insights, AI empowers managers to make more informed decisions about quality control measures.
To fully harness the power of AI for quality incident management, manufacturers should consider the following strategies:
- Invest in robust data collection and management systems to ensure AI has access to high-quality, relevant data.
- Foster a culture of data-driven decision-making throughout the organization.
- Provide ongoing training to staff to ensure they can effectively utilize AI-powered tools.
- Regularly review and update AI models to ensure they remain accurate and relevant.
- Integrate AI-driven RCA with other quality management processes for a holistic approach to quality control.
By embracing these revolutionary improvements, manufacturers can transform their approach to quality incident management. The result is better handling of quality issues and a fundamental shift towards more proactive, efficient, and effective quality control. As AI continues to evolve, its potential to drive improvements in manufacturing quality will only grow, making it an essential tool for any forward-thinking manufacturer.
Tagged:
Thinking about Botable for your team? See it live
.png)
In just 30 minutes, see how Botable can transform your workplace with a personalized demo.
Identify your unique challenges
Flexible pricing options
Easy integrations
Step-by-step implementation plan
Customize Botable for your workflow
Meet with the team



.png)