Building an AI chatbot for Quality Management Systems
As we delve deeper into the world of AI chatbots for quality management systems, we'll explore their key advantages and critical differences from traditional chatbots and provide a step-by-step guide on building and implementing these powerful tools in your organization's quality management framework.
Organizations are turning to cutting-edge technologies such as generative AI to streamline processes and enhance efficiency. Enter AI chatbots – the game-changing solution revolutionizing quality management systems (QMS). This expert guide will walk you through the intricate process of building an AI chatbot tailored for QMS, unlocking new productivity levels and precision in your quality control efforts. Whether you're a quality manager, a tech enthusiast, or a business leader looking to stay ahead of the curve, this comprehensive walkthrough will equip you with the knowledge and insights needed to harness the power of AI in your quality management journey.
Introduction to AI Chatbots in Quality Management Systems
Defining AI Chatbot and Its Role in Enhancing Quality Management
An AI chatbot is a sophisticated software application that simulates human-like conversations through text or voice interactions. In the context of quality management systems, these intelligent virtual assistants are potent tools for enhancing quality control and assurance processes. By leveraging artificial intelligence and machine learning algorithms, AI chatbots can analyze vast data, provide real-time insights, and offer personalized solutions to quality-related queries and issues.
The role of AI chatbots in enhancing quality management is multifaceted. They act as tireless, round-the-clock assistants, capable of handling routine inquiries, guiding users through complex quality procedures, and even predicting potential quality issues before they arise. This constant availability ensures that quality management processes remain efficient and responsive, regardless of time or resource constraints.
Moreover, AI chatbots excel at data collection and analysis, a crucial aspect of any robust quality management system. They can gather information from various sources, identify patterns, and generate reports that help decision-makers make informed choices about quality improvement initiatives. This data-driven approach leads to more accurate quality assessments and effective corrective actions.
The Evolution of Chatbots to AI-Powered Solutions
The journey from simple chatbots to AI-powered solutions in quality management systems represents a significant leap in technological capability. Early chatbots were rule-based systems with limited functionality, often restricted to answering predefined questions with scripted responses. While useful for basic tasks, these early iterations lacked the flexibility and intelligence required for complex quality management processes.
As artificial intelligence and machine learning technologies advanced, so did chatbots' capabilities. The transition to AI-powered solutions marked a turning point in their application to quality management systems. These new-generation chatbots could understand context, learn from interactions, and provide more nuanced and accurate responses to user queries.
The evolution brought about several key improvements:
- Natural Language Processing (NLP): AI chatbots can now understand and interpret human language more accurately, allowing for more natural and fluid conversations.
- Machine Learning: These systems can learn from each interaction, continuously improving their responses and adapting to new quality management challenges.
- Predictive Analytics: AI-powered chatbots can analyze trends and predict potential quality issues, enabling proactive quality management.
- Integration Capabilities: Modern AI chatbots can seamlessly integrate with existing quality management tools and databases, providing a more holistic approach to quality control.
This evolution has transformed AI chatbots from simple query-response tools to intelligent assistants capable of handling complex quality management tasks. They now play a crucial role in streamlining processes, reducing human error, and enhancing overall quality management efficiency.
As we delve deeper into the world of AI chatbots for quality management systems, we'll explore their key advantages, the critical differences from traditional chatbots, and provide a step-by-step guide on how to build and implement these powerful tools in your organization's quality management framework.
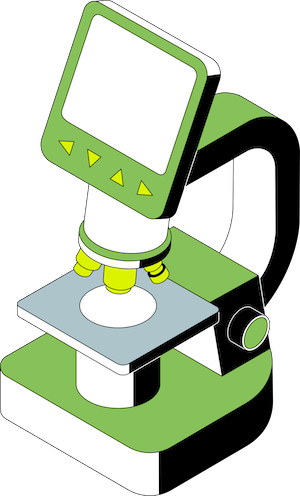
Key Advantages of Integrating AI Chatbots into Quality Management Systems
Real-Time Feedback and Continuous Improvement
One of the most significant advantages of integrating AI chatbots into quality management systems is their ability to provide real-time feedback and facilitate continuous improvement. AI-powered quality prediction tools can analyze vast amounts of data to predict potential issues and take proactive measures to address them before products reach the market. This predictive capability allows organizations to stay ahead of quality concerns, reducing the risk of defects and improving overall product quality.
Moreover, AI chatbots can continuously learn from interactions and update their knowledge base, ensuring that the quality management system evolves with the organization's needs. This adaptive nature supports a culture of continuous improvement, where quality processes are constantly refined based on real-world data and experiences.
Automation of Routine Quality Checks and Reporting
AI chatbots excel at automating routine quality checks and reporting, freeing up human resources for more complex tasks. AI-powered tools can monitor production processes in real time to identify defects and quality issues, reducing the risk of defects and improving overall product quality. This constant vigilance ensures that quality standards are consistently met without the need for constant human oversight.
Furthermore, AI chatbots can generate detailed reports on quality metrics, trends, and anomalies with minimal human intervention. This automation not only saves time but also reduces the likelihood of human error in data collection and analysis, leading to more accurate and reliable quality management processes.
{{cta}}
Personalized Solutions for Quality Management Challenges
AI chatbots analyze individual user interactions and historical data to offer personalized solutions to quality management challenges. They can tailor their responses and recommendations based on the specific context of each query, providing more relevant and effective solutions to quality-related issues.
AI-driven QM solutions can assess the sentiment in every customer interaction, allowing contact center managers to proactively address quality issues instead of merely identifying them. This level of personalization extends beyond customer interactions to internal quality management processes, where AI chatbots can offer customized guidance to employees based on their role, experience, and past performance in quality-related tasks.
Data-Driven Insights for Decision Making
Perhaps one of the most potent advantages of AI chatbots in quality management systems is their ability to provide data-driven insights for decision-making. By analyzing vast amounts of quality-related data, AI chatbots can identify patterns, trends, and correlations that might be invisible to human observers.
Automated workflows and AI-assisted scoring can enhance the consistency and thoroughness of quality analysts' work, enabling them to better detect trends and potential quality issues. These insights empower decision-makers to make more informed choices about quality improvement initiatives, resource allocation, and strategic planning.
AI chatbots can also predict future quality trends based on historical data and current conditions, allowing organizations to proactively maintain or improve quality standards. This predictive capability is precious in industries where quality issues can have significant financial or safety implications.
By leveraging these key advantages, organizations can transform their quality management systems into more efficient, responsive, and effective tools for ensuring product and service excellence. Integrating AI chatbots not only streamlines quality processes but also provides a competitive edge in today's data-driven business landscape.
Critical Differences Between Traditional and AI-Enhanced Chatbots
As we delve into the world of chatbots for quality management systems, it's crucial to understand the fundamental differences between traditional chatbots and their AI-enhanced counterparts. These differences highlight the technological advancements and underscore the superior capabilities that AI brings to quality management processes.
Smart Learning Capabilities: From Predefined Responses to Predictive Assistance
Traditional chatbots operate on a set of predefined rules and responses. They function like a sophisticated decision tree, where each user input triggers a specific, pre-programmed response. While effective for simple, routine queries, these chatbots struggle with complex or nuanced questions that fall outside their programmed scope.
In contrast, AI-enhanced chatbots leverage machine learning algorithms to improve their understanding and responses continuously. These innovative learning capabilities allow AI chatbots to:
- Adapt to new information and scenarios without manual reprogramming
- Understand the context and intent behind user queries, leading to more accurate responses
- Learn from past interactions to improve future responses
- Identify patterns in quality-related issues and predict potential problems before they occur
This evolution from rigid, predefined responses to dynamic, predictive assistance marks a significant leap in chatbot technology. In quality management systems, this translates to more efficient problem-solving, proactive issue identification, and continuous improvement of quality processes.
Personalization Through Interaction Analysis and User Behavior
Traditional chatbots offer a one-size-fits-all approach, treating each user interaction as isolated and independent. They cannot remember past conversations or adapt their communication style to individual users.
AI-enhanced chatbots, on the other hand, excel at personalization through sophisticated interaction analysis and user behavior tracking. These advanced chatbots can:
- Remember past interactions with specific users, providing context-aware responses
- Analyze user behavior patterns to anticipate needs and preferences
- Tailor their communication style to match the user's level of expertise and role within the quality management system
- Offer personalized recommendations based on the user's history and current context
This personalization capability is invaluable in quality management. It allows the AI chatbot to provide targeted assistance to stakeholders—from quality control inspectors to senior management—ensuring that each user receives the most relevant and helpful information for their specific needs.
Moreover, by analyzing interaction patterns across multiple users, AI chatbots can identify common quality issues, frequently asked questions, and areas of confusion within the quality management system. This insight can improve training programs, documentation, and overall quality processes.
The shift from generic, rule-based interactions to personalized, context-aware assistance represents a significant advancement in chatbot technology for quality management systems. It enables more efficient problem-solving, enhances user satisfaction, and contributes to more effective quality management practices.
As we continue to explore the implementation of AI chatbots in quality management systems, these critical differences between traditional and AI-enhanced chatbots will play a crucial role in shaping the future of quality control and assurance processes.

Step-by-Step Guide on Building an AI Chatbot for Quality Management
Building an AI chatbot for quality management systems is complex but rewarding. This step-by-step guide will walk you through the essential stages of AI chatbot development, ensuring you create a powerful tool that enhances your quality management processes.
Identifying the Core Objectives for the AI Chatbot within a QMS
The first step in creating an AI chatbot for quality management is clearly defining its objectives. This involves:
- Analyzing your current QMS processes to identify areas that could benefit from automation
- Determining the critical pain points that users face when interacting with the QMS
- Setting specific, measurable goals for the chatbot (e.g., reducing response time for quality-related queries by 50%)
- Prioritizing features based on their potential impact on quality management efficiency
Establishing clear objectives lays the foundation for a focused and effective ai chatbot development process.
Selecting the Right Technology Stack and Platforms
Choosing the appropriate technology is crucial when building an ai chatbot. Consider the following factors:
- Natural Language Processing (NLP) capabilities to ensure accurate understanding of user queries
- Machine Learning frameworks that allow the chatbot to improve over time
- Integration capabilities with your existing QMS software and databases
- Scalability to handle growing volumes of interactions and data
- Security features to protect sensitive quality management information
Curating Content and Knowledge Base Tailored for Quality Management Needs
A robust knowledge base is the backbone of any effective AI chatbot. When building AI chatbots for quality management:
- Compile a comprehensive database of quality management processes, standards, and best practices
- Include frequently asked questions and their detailed answers
- Incorporate real-world examples and case studies to provide context
- Ensure the content is up-to-date with the latest quality management standards and regulations
Remember to structure this information in a way that's easily accessible and understandable for the AI chatbot.
Designing Conversational Flows Aimed at Streamlining QMS Processes
Effective conversation design is critical to creating an AI chatbot that enhances QMS processes. Consider these steps:
- Map out everyday user journeys within your quality management system
- Design conversation flows that guide users through these journeys efficiently
- Create decision trees to handle various scenarios and user inputs
- Incorporate prompts and suggestions to help users navigate complex quality management tasks
- Ensure the chatbot can seamlessly hand over to human agents when necessary
The goal is to create intuitive, natural conversations that streamline quality management processes and improve user experience.
Incorporating Feedback Loops for Continuous Learning and Adaptation
To ensure your AI chatbot continues to improve over time, implement robust feedback mechanisms:
- Collect user feedback after each interaction
- Analyze conversation logs to identify areas for improvement
- Implement A/B testing for different conversational approaches
- Regularly update the chatbot's knowledge base with new information and insights
- Use machine learning algorithms to refine the chatbot's responses based on user interactions continuously
By incorporating these feedback loops, you ensure your AI chatbot evolves alongside your quality management needs, becoming more effective and efficient.
Building an AI chatbot for quality management is an iterative process that requires careful planning, execution, and ongoing refinement. By following these steps and focusing on the specific needs of your quality management system, you can create a powerful tool that significantly enhances your QMS processes and overall quality control efforts.
Harnessing Advanced Technologies in Developing AI Chatbots for QMS
The development of AI chatbots for Quality Management Systems (QMS) is at the forefront of technological innovation. These chatbots are revolutionizing how organizations approach quality control and management by leveraging cutting-edge technologies. Let's explore the key advanced technologies shaping AI chatbots' future in QMS.
Natural Language Processing (NLP) for Enhanced Understanding
Natural Language Processing (NLP) is a cornerstone technology in developing sophisticated AI chatbots for QMS. NLP enables chatbots to understand and interpret human language in its natural form, bridging the gap between machine comprehension and human communication. In the context of quality management, NLP allows chatbots to:
- Accurately interpret complex quality-related queries from users
- Understand the context and intent behind questions, leading to more relevant responses
- Process unstructured data from various sources, such as customer feedback or quality reports
- Generate human-like responses that are easy for users to understand and act upon
By harnessing the power of NLP, AI chatbots can provide more intuitive and effective support for quality management processes, enhancing user experience and streamlining communication within the QMS.
Employing Machine Learning Algorithms for Predictive Quality Control
Machine Learning (ML) algorithms are transforming the landscape of quality control by enabling AI chatbots to predict and prevent quality issues before they occur. These algorithms analyze vast amounts of historical and real-time data to identify patterns and trends that might be invisible to human observers. In QMS, ML-powered chatbots can:
- Predict potential quality issues based on historical data and current production parameters
- Recommend preventive actions to maintain quality standards
- Continuously learn from new data and improve their predictive accuracy over time
- Adapt to changing quality requirements and production conditions
Around 75 percent of the value derived from generative AI use cases spans areas including customer operations, which can enhance customer care functions by 30-45 percent through automation, intelligence, and learning. This statistic underscores the significant impact that ML-powered AI chatbots can have on quality management processes, particularly in customer-facing aspects of quality control.
Leveraging Big Data Analytics for Problem-Solving Insights
Big Data Analytics is another crucial technology in developing AI chatbots for QMS. These chatbots can provide valuable insights for problem-solving and decision-making in quality management by processing and analyzing large volumes of structured and unstructured data. The application of Big Data Analytics in QMS chatbots includes:
- Analyzing quality metrics across multiple production lines or facilities to identify best practices
- Correlating customer feedback with production data to pinpoint areas for quality improvement
- Identifying root causes of quality issues by examining diverse data sources
- Generating comprehensive reports that help quality managers make data-driven decisions
Combining Big Data Analytics with AI chatbots creates a powerful tool for quality management, enabling organizations to harness the full potential of their data for continuous quality improvement.
By integrating these advanced technologies - NLP, Machine Learning, and Big Data Analytics - into AI chatbots, organizations can create sophisticated quality management systems that are more responsive, predictive, and insightful. These AI-powered chatbots streamline quality control processes and contribute to a culture of continuous improvement and data-driven decision-making in quality management.
As these technologies evolve, we can expect AI chatbots to play an increasingly central role in quality management systems, driving efficiency, accuracy, and innovation in quality control practices across industries.
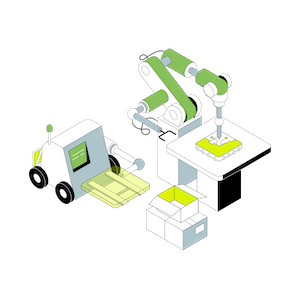
Best Practices in Deploying AI Chatbots within Quality Management Systems
Implementing AI chatbots in Quality Management Systems (QMS) can significantly enhance efficiency and effectiveness. However, successful deployment requires careful planning and adherence to best practices. Let's explore the key considerations for integrating AI chatbots into your QMS.
The Importance of Stakeholder Engagement and Change Management
Introducing AI chatbots into a QMS represents a significant change affecting various organizational stakeholders. Effective stakeholder engagement and change management are crucial for successfully implementing generative AI chatbots. Consider the following approaches:
- Identify key stakeholders and involve them early in the planning process
- Communicate the benefits of AI chatbots clearly to all affected parties
- Address concerns and resistance proactively through open dialogue and education
- Provide comprehensive training to ensure the smooth adoption of the new technology
84% of companies believe that AI chatbots will become more important for communication between customers and businesses. This statistic underscores the growing significance of AI chatbots and can be used to build a compelling case for stakeholder buy-in.
Maintaining Data Security and User Privacy
When deploying AI chatbots in QMS, data security and user privacy should be top priorities. These systems often handle sensitive quality-related information, making it crucial to implement robust security measures:
- Ensure compliance with relevant data protection regulations (e.g., GDPR, CCPA)
- Implement strong encryption for data in transit and at rest
- Regularly conduct security audits and vulnerability assessments
- Establish straightforward data handling and retention policies
- Provide transparency to users about how their data is collected, used, and protected
By prioritizing data security and user privacy, you can build trust in the AI chatbot system and encourage its adoption within the QMS.
Ongoing Training and Development Strategies
To maximize the effectiveness of AI chatbots in QMS, it's essential to implement ongoing training and development strategies:
- Regularly update the chatbot's knowledge base with the latest quality management practices and standards
- Continuously train the AI model using real-world interactions to improve its accuracy and relevance
- Implement a feedback loop where users can report issues or suggest improvements
- Conduct periodic reviews of chatbot performance and identify areas for enhancement
Remember that AI chatbot development is an iterative process. Continuous improvement ensures that the chatbot remains a valuable asset to your QMS.
Success Metrics: Evaluating the Impact on QMS Efficiency
To justify the investment in AI chatbots and guide future improvements, it's crucial to establish and monitor success metrics. Consider the following key performance indicators (KPIs):
- Response time to quality-related queries
- Accuracy of chatbot responses
- User satisfaction ratings
- Reduction in human intervention for routine quality management tasks
- Impact on overall quality metrics (e.g., defect rates, customer satisfaction)
90% of businesses report significant improvements in the speed of complaint resolution due to the use of chatbots. This statistic highlights the potential impact of AI chatbots on QMS efficiency and can serve as a benchmark for your implementation.
When evaluating the impact of AI chatbots on QMS efficiency, consider both quantitative metrics and qualitative feedback from users. This comprehensive approach will provide a holistic view of the chatbot's performance and contribution to your quality management efforts.
By following these best practices, organizations can effectively deploy AI chatbots within their quality management systems, improving efficiency, making better decisions, and enhancing overall quality control. As AI technology evolves, staying informed about the latest developments and continuously refining your implementation strategy will ensure that your QMS remains at the forefront of innovation.
Common Challenges and Solutions in Implementing AI Chatbots for QMS
Implementing AI chatbots in quality management systems (QMS) offers numerous benefits, but organizations often face several challenges. Understanding these hurdles and having strategies to overcome them is crucial for successful implementation. Let's explore some common challenges and their potential solutions.
Addressing Integration Hurdles with Existing QMS Software
One of the primary challenges in implementing AI chatbots for QMS is integrating them seamlessly with existing software systems. Many organizations have established QMS platforms that may not be immediately compatible with new AI technologies. To address this challenge:
- Conduct a thorough assessment of your current QMS infrastructure to identify potential integration points and limitations.
- Choose AI chatbot solutions that offer robust API capabilities for easier integration with existing systems.
- Consider implementing middleware solutions that can act as a bridge between the AI chatbot and legacy QMS software.
- Work closely with your IT department and the chatbot vendor to develop custom integration solutions if necessary.
- Plan a phased implementation approach, starting with less critical processes to minimize disruption.
By proactively addressing these integration hurdles, you can ensure that your AI chatbot becomes a seamless part of your existing QMS ecosystem, enhancing rather than disrupting your quality management processes.
Overcoming Resistance to Change among Employees
Introducing AI chatbots into QMS processes can often meet resistance from employees accustomed to traditional methods. This resistance can stem from fear of job displacement, lack of understanding about AI technology, or concerns about the chatbot's reliability. To overcome this challenge:
- Communicate clearly and transparently about the purpose and benefits of implementing AI chatbots in the QMS.
- Provide comprehensive training programs to help employees understand how to work alongside AI chatbots effectively.
- Emphasize that AI chatbots are tools to augment human capabilities, not replace them.
- Involve employees in the implementation process, seeking their input and feedback to create a sense of ownership.
- Showcase early successes and positive outcomes to build confidence in the new system.
- Offer ongoing support and resources to help employees adapt to the new technology.
Addressing employee concerns and fostering a culture of collaboration between humans and AI can significantly reduce resistance and promote the adoption of AI chatbots in your QMS.
Navigating Limitations in Technological Infrastructure
Implementing AI chatbots often requires robust technological infrastructure, including high-speed internet, sufficient computing power, and adequate data storage capabilities. Organizations with limited technological resources may face challenges in this area. To navigate these limitations:
- Conduct a thorough assessment of your current technological infrastructure to identify gaps and needs.
- Consider cloud-based AI chatbot solutions that can reduce the burden on your local infrastructure.
- Prioritizing infrastructure upgrades is critical for AI chatbot implementation, such as improving network capacity or enhancing data storage capabilities.
- Explore partnerships with technology providers who can offer scalable solutions tailored to your organization's needs and growth plans.
- Implement a phased approach to infrastructure upgrades, aligning them with the gradual rollout of AI chatbot capabilities in your QMS.
Addressing these technological limitations strategically allows you to create a solid foundation for implementing and scaling AI chatbots within your QMS, even with initial resource constraints.
Overcoming these common challenges requires strategic planning, clear communication, and technological adaptability. By anticipating these hurdles and preparing appropriate solutions, organizations can smooth the path for successfully implementing AI chatbots in their Quality Management Systems, ultimately leading to improved efficiency and effectiveness in quality control processes.

Future Trends: The Evolving Role of AI Chatbots in Quality Management
As technology advances rapidly, generative AI chatbots' role in quality management is set to evolve significantly. Understanding these future trends can help organizations stay ahead of the curve and maximize the potential of AI in their quality management systems.
Anticipated Advances in Artificial Intelligence and Their Implications
The field of artificial intelligence is constantly progressing, and several anticipated advances are likely to have profound implications for AI chatbots in quality management:
- Enhanced Natural Language Processing (NLP): Future AI chatbots will likely possess more sophisticated NLP capabilities, allowing them to understand and respond to complex quality-related queries with greater accuracy and nuance. This advancement will enable more natural and productive interactions between users and chatbots.
- Emotional Intelligence: AI chatbots may develop the ability to recognize and respond to human emotions. In quality management, this could lead to more empathetic handling of customer complaints and more effective communication with team members during quality control processes.
- Autonomous Decision Making: As AI algorithms become more advanced, chatbots may gain the ability to make autonomous decisions in specific quality management scenarios. This could include initiating quality control measures or adjusting production parameters based on real-time data analysis.
- Multimodal Interaction: Future AI chatbots may be able to process and respond to multiple input types, including text, voice, and even visual data. This could revolutionize quality inspections and reporting processes, allowing for more comprehensive and efficient quality management.
- Explainable AI: As AI chatbots take on more critical roles in quality management, there will be a growing need for "explainable AI" - systems that can provide clear reasoning for their decisions and recommendations. This transparency will maintain trust and accountability in quality management processes.
These advancements will likely lead to AI chatbots becoming even more integral to quality management systems, potentially taking on roles previously thought to require human judgment and expertise.
The Growing Significance of Real-Time Data Analytics in Quality Assurance
Real-time data analytics is set to play an increasingly crucial role in quality assurance, with AI chatbots at the forefront of this trend:
- Predictive Quality Control: AI chatbots equipped with advanced analytics capabilities can predict quality issues before they occur. By analyzing real-time data from production processes, supply chains, and customer feedback, these chatbots can alert quality managers to potential problems and suggest preventive actions.
- Continuous Monitoring: Future AI chatbots will likely offer round-the-clock quality metrics monitoring across all production and service delivery stages. This constant vigilance will enable immediate detection and response to quality deviations, significantly reducing the risk of defects reaching customers.
- Personalized Quality Insights: As AI chatbots become more adept at processing and analyzing large volumes of data, they can provide highly customized quality insights to different stakeholders. For example, a production manager might receive real-time updates on manufacturing quality, while a customer service representative gets instant access to product quality history for a specific customer.
- Integration with IoT Devices: Integrating AI chatbots with Internet of Things (IoT) devices will enable more comprehensive data collection and analysis. This could lead to more accurate quality assessments and faster identification of the root causes of quality issues.
- Dynamic Quality Standards: With real-time data analytics, AI chatbots could help organizations implement dynamic quality standards that adapt to changing conditions. For instance, quality thresholds could be automatically adjusted based on factors like raw material variations or environmental conditions.
The growing significance of real-time data analytics powered by AI chatbots will likely lead to more proactive and adaptive quality management practices. Organizations that embrace these technologies will be better positioned to maintain high-quality standards in increasingly complex and fast-paced business environments.
As these trends continue to unfold, it's clear that AI chatbots will play an increasingly central role in shaping the future of quality management. Organizations that stay informed about these developments and proactively integrate advanced AI capabilities into their quality management systems will be well-positioned to maintain a competitive edge in their respective industries.
Maximizing the Potential of AI Chatbots in Enhancing QMS Efficacy
Organizations need to adopt a strategic approach to their development and implementation to fully leverage the power of AI chatbots in Quality Management Systems (QMS). By following best practices for creating AI chatbot solutions tailored for QMS, companies can significantly enhance their quality control processes and overall efficiency.
- Define Clear Objectives: Before diving into how to build an AI chatbot for QMS, it's crucial to define clear objectives. Identify areas within your quality management processes that could benefit from automation and AI assistance. AI-powered chatbots can provide instant feedback and support to employees, streamlining communication and helping employees access information needed for data-driven decisions. This capability can be a guiding principle in setting your chatbot's objectives.
- Choose the Right Development Approach: When considering how to make AI chatbot for QMS, you have several options:
- Use existing chatbot platforms with AI capabilities
- Develop a custom solution from scratch
- Employ a hybrid approach combining pre-built components with custom elements
The choice depends on your specific needs, in-house expertise, and resources. For those wondering how to build a chatbot from scratch, this approach offers the most flexibility but requires significant technical expertise and time investment.
- Natural Language Processing (NLP): A key aspect of developing a chatbot for QMS is ensuring it can understand and respond effectively to natural language queries. Invest in robust NLP capabilities to enable your chatbot to interpret complex quality-related questions and provide accurate, context-aware responses.
- Integrate with Existing QMS Infrastructure: Ensure your AI chatbot seamlessly integrates with your existing QMS software and databases. This integration is crucial for providing real-time, accurate information and executing quality-related tasks efficiently.
- Implement Continuous Learning Mechanisms: To maximize the potential of your AI chatbot, implement mechanisms for continuous learning and improvement. This involves:
- Regular updates to the knowledge base
- Analysis of user interactions to identify areas for improvement
- Incorporation of feedback from quality management teams
- Prioritize User Experience: When considering building an AI chatbot for QMS, user experience should be a top priority. Design intuitive conversational flows that guide users through quality management processes effectively. Chatbots can resolve 58% of returns and cancellations, and 61% of respondents believe chatbots can boost productivity by automating task follow-ups. This statistic underscores the importance of creating user-friendly chatbot interfaces that handle various quality-related queries and tasks.
- Leverage Advanced Analytics: Incorporate advanced analytics capabilities into your AI chatbot to provide deeper insights into quality trends and potential issues. This can help in predictive quality management and proactive problem-solving.
- Ensure Scalability and Flexibility: As you develop your AI chatbot, ensure it's designed to scale with your organization's growth and adapt to changing quality management needs. This might involve using cloud-based solutions or modular architectures allowing easy expansion and modification.
- Implement Robust Security Measures: Given the sensitive nature of quality management data, implementing strong security measures is crucial. Ensure your AI chatbot adheres to data protection regulations and employs encryption and secure authentication.
- Measure and Optimize Performance: Regularly assess your AI chatbot's performance against key metrics such as response accuracy, user satisfaction, and impact on quality indicators. Use these insights to refine and improve your chatbot's capabilities continuously.
By following these strategies, organizations can maximize the potential of AI chatbots in enhancing QMS efficacy. Businesses using AI chatbots have 3x better conversion into sales than those using website forms, and generative AI can increase conversion into sales by 2.5x. While this statistic is focused on sales, it illustrates AI chatbots' significant impact on business processes, including quality management.
In conclusion, learning how to create AI chatbot solutions for QMS is an investment that can yield substantial returns in terms of improved quality control, increased efficiency, and enhanced decision-making capabilities. By carefully planning and executing your AI chatbot strategy, you can transform your quality management processes and gain a competitive edge in today's data-driven business landscape.
Tagged:
Answers your employees need, right when they need them
Meet Botable — the AI chatbot that handles everything from simple FAQs to complex, multi-step questions, so your team can focus on what matters. Built for HR, QA, and beyond.
Continue reading
Ready to see what Botable can do for you?
Book your demo now to see how Botable can transform your workplace.
Identify your unique challenges
Flexible pricing options
Easy integrations
Step-by-step implementation plan
Customize Botable for your workflow
Book a demo
.webp)